Modern Face Recognition Systems
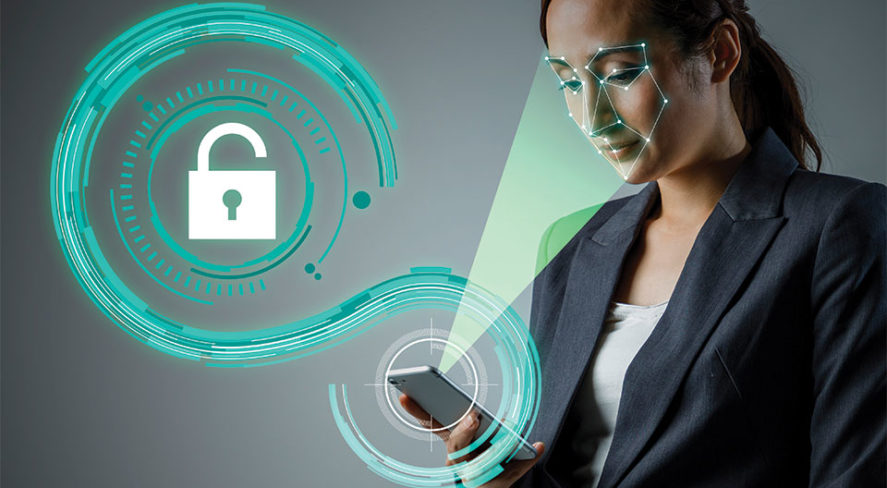
Living the revolution as algorithms based on Deep Neural Networks (DNN) come to market
Face recognition as a technology experienced its first wave of adoption in 2000s, mostly in government projects. We must admit that the quality and performance of the previous generation of face recognition products simply doesn’t hold up against criticism, although many global vendors introduced face recognition as newly created solutions (using available third-party face recognition engines) or rose to success with their own face recognition technology.
The limitations of such legacy technology and systems will affect government agencies around the world for another 10 years (at least) since they both can’t immediately give up already implemented and supported systems. That would basically be admitting the expenditure of hundreds of millions of dollars on technology of questionable quality. In addition, agencies face a stumbling block in the lack of a legal framework setting minimal requirements for the face recognition engines/systems.
Luckily, commercial businesses that have tried face recognition for their tasks during the first wave of adoption quickly learned that the technology was not there yet to bring measurable value to their day-to-day operations. And it was for a very solid reason: Businesses didn’t have a need nor the resources for sophisticated suspect profile investigation software (for investigating a customer or employee in their case) with a variety of manual tools for face image treatment or ISO standard-based reports.
As business itself went digital, the need to interact with customers and employees in an interconnected environment allowing contactless person identification (which face recognition enables) has led to the concept of cross-platform and cross-domain face recognition.
Breakthroughs in the approach to face recognition happened in 2012 with the introduction of deep neural networks (DNN)-based algorithms that now penetrate the full-face recognition pipeline with state-of-the-art products. We are entering the second wave of face recognition technology adoption, now driven by commercial businesses willing to give the technology a second chance.
Imagine modern business working today through the following multiple channels where it interacts with employees and customers, who are typically divided into controlled or uncontrolled scenarios:
Controlled (user interacts with the system in the process of acquiring the best shot of the face for recognition)
- Mobile
- Web (including web-based software)
- Standalone devices (digital signage)
Uncontrolled (no interaction/cooperation of the user required to capture the best shot of the face for recognition)
- IP camera surveillance (doesn’t have to be security-oriented)
Having the seamless customer/employee journey across all the domains described above is the key for any business case of modern enterprises. This, in turn, dictates a set of “must-haves” facing recognition engines and solutions providers. Let’s have a look at them:
Mobile
- Work with mobile camera stream for face detection/best shot selection (no selfies anymore)
- Support offline 1:1 face matching with the acceptable face recognition speed (less than 500ms)
- Have liveness (anti-spoofing) algorithm
- Have stable performance across a variety of at least iOS- and Android-based devices
Web
- Work in JavaScript with no performance decrease
- Have liveness (anti-spoofing) algorithm
- Standalone devices
- Work with commonly available web cameras
- Support both 1:1 and 1:N face matching with the acceptable face recognition speed (less than 500ms)
- Have stable performance across a variety of embedded system on chips
IP camera surveillance
- No special “special face recognition camera” acceptable: steady demand for the use of the existing infrastructure
- Ability to implement modular system, separating frontend and backend modules
- Ability to handle thousands of camera channels and thousands of face identification requests per second with rational backend requirement
Now, we see that both engine and the solution must be versatile enough to operate on various platforms and across many use cases. The reality is that today face recognition solutions are being used by several business divisions of companies pursuing various goals as a part of an overall user experience. However, the system must be unified to enable these different use cases and business logic. And companies will not consider launching a separate project every time they want to add the new domain for using face recognition as an enabling person identification technology.
The typical workflow and gradual face recognition technology implementation could go many possible ways with simultaneous introduction of subsequent new features, mixing functionality, like the example described here:
- Step 1: A company decides to introduce mobile authentication function to the customer app, and initial face data for enrollment will be gathered through the mobile app and at the remote office web camera at the service desk.
- Step 2: The company decides to track customers in their remote offices using IP camera; the previously enrolled face data from the mobile app or remote office web camera at the service desk should be used to enable this function.
- Step 3: The next feature would be adding depersonalized analytics of the people flow in the remote offices: age, gender, race, emotions.
- Step 4: Other features could include a time-and-attendance solution for employees either through an IP camera or standalone devices (could be mobile devices as well).
- Step 5: Finally, the company would like to track and measure the conversion of the unidentified visitors of the branch office to the enrolled customers in a database.
While the use cases may be straightforward, traditional face recognition solution vendors would find it challenging to build up such new functionality on top of an existing database or users, or dynamically changing a database of prospective customers, leading to parallel operation of face recognition engines that meet the expectations of performance and quality of each business unit. That brings us to the most important part of this article: How to make sure that cross-domain face recognition is possible with a specific face recognition solution vendor or engine?
The best way to ensure that a face recognition engine would cover most of your evolving business needs is to refer to “The Ongoing Face Recognition Vendor Test” collated by the U.S. National Institute of Standards and Technology (NIST) and see the performance of algorithms listed there across such domains as visa, webcam, selfie, mugshot and wild (uncontrolled). You should pay attention to the most stable (leveled) top results of a vendor across all categories.
There is another set of face recognition engine parameters to pay attention to that directly influence the performance: face template size. There needs to be a balance between the face template size and the achieved quality across domains. Face template creation time directly affects the ability of an algorithm to run on a mobile device in offline mode. Take care to compare times. The algorithm may be tops in all categories but 100 times slower than its competitors.
State-of-the-art face recognition algorithms are evolving fast and aim for the mass adoption in many industries in the near term. The only suggestion for any business would be to closely monitor available trusted resources for algorithms evaluation, keeping in mind that more and more business units are becoming interested in face recognition as an enabling person identification technology. A key competitive advantage in businesses with a rapidly changing technology landscape is adaptability, so flexible face recognition technology that efficiently meets most business cases is a must.
Alexander Khanin is CEO at Vision Labs.