Enhancing Vehicle Inspections with Artificial Intelligence
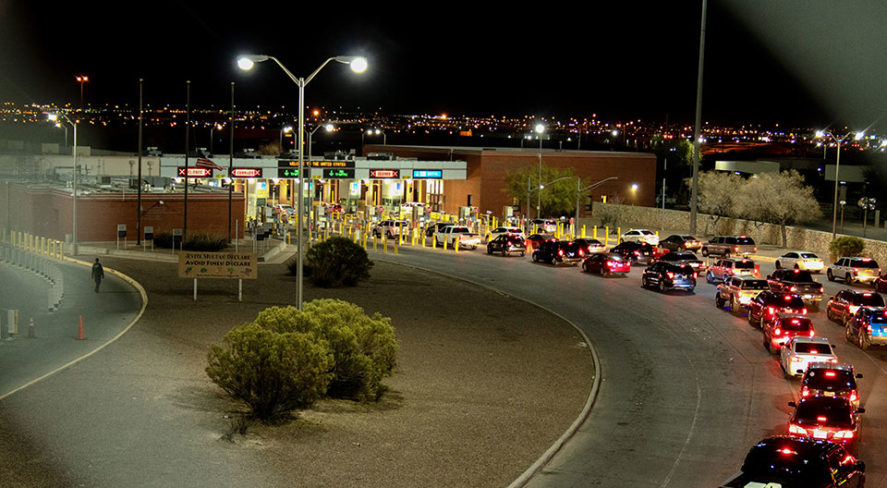
Computer vision “sees” details that can match cars to a database and identify threats
Technology offers new ways to close the gap in how we approach security challenges. Vehicle inspection and identification no longer have to rely solely on guards using mirrors and observing license plates. Artificial intelligence (AI) and automation can do more with existing technologies, empowering security professionals to do their jobs faster and more accurately.
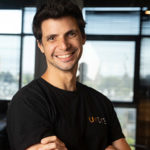
Sensitive facilities and secured building compounds rely on license plate recognition at vehicle access control points, but hundreds of license plates are fraudulently copied and used every month in the United States alone, and using a fake or stolen license plate can easily breach a perimeter’s security. Criminals and terror groups around the world have already done this for robberies, murders, planting of illicit materials, and more.
So if a license plate is such a sensitive asset, why is it not more secure and why are there not more methods to cross-reference and identify a vehicle?
Solutions to prevent stolen license plates include non-reliable or manual means like strengthening the screws of the license plate holder, but they still rely on only one source of verification.
Using AI and computer vision can help resolve some of these issues. In addition, inspecting other elements of the vehicle, such as the undercarriage, may help with the process of identifying a vehicle and its occupants in a more secure manner. Methods like deep learning and segmentation of images can help to categorize certain areas and tag them with parameters that are understood and recorded by the AI.
Computer vision is an interdisciplinary AI field that is concerned with how computers view and understand digital images and videos. This includes all tasks performed by biological vision systems, including sensing a visual stimulus, understanding what it is seeing, and extracting complex information onto a form that can be applied to other processes. It simulates elements of human vision by using sensors, computers and machine learning algorithms. Although computers cannot see the same way humans can, modern computer vision technology can surpass human vision capabilities in some regards.
Computer vision can be applied to many fields, including the medical, automotive, security, retail and commercial industries. There are many real-world examples because computer vision theory can be applied to any use case in which a computer “sees” its surroundings in some form. Autonomous driving relies on image recognition so that the vehicle can avoid crashing into other cars and obstacles.
In order to give the computer vision algorithm the best chance to analyze an image correctly, superior-quality, durable hardware is needed to present the same type of high-resolution image time after time. An undercarriage is a dirty and difficult area to inspect that can produce many false positives, so there is no way to compromise on this.
When looking at the undercarriage of a vehicle after obtaining a high-resolution scan, there are many parameters that can be used to develop a unique signature. Elements such as screws, their placement and even angle, broken parts, rust, and positioning of components such as the fuel tank or exhaust pipe can help to identify and verify a vehicle.
Different sub-models of vehicles might have slight differences in the way their undercarriages are assembled. While two vehicles could look completely identical in an overview image, their undercarriages can have subtle variations. Without added layers of security in place, it is very easy for bad actors to get hold of a recurring vehicle list, produce an identical license plate, and place it on a vehicle of the same make and model.
Undercarriage verification is similar to face verification. The objective is to create a decision system that identifies the undercarriage of cars of the same model and provides a similarity score.
When viewing an image of an undercarriage, the AI segments each component and area and classifies each of its parts. Once it has seen a certain part enough times, it will know how it is actually supposed to look and will analyze every image it is shown.
In order to produce a highly accurate method of identification, the system combines three indicators, including:
- Vehicle undercarriage unique identity markers – a collection of multiple visual features, encoded for instance separation
- Overview image, providing coarse markers, including brand, model, color. and plastic components
- License plate or VIN – the vehicle identifier for the database
The result is a quick analysis that produces a compatibility score by comparing the vehicle in question to the vehicle in the database that has the same license plate. Once the system has matched the two undercarriage images and other elements, a user is able to determine whether it is the same vehicle or not.
Identification methods such as these can provide an extra layer of security to facilities worldwide, including airports, seaports, embassies, energy plants, prisons, border control sites, military bases, hotels, casinos, stadiums and more.
Examination of undercarriages goes beyond vehicle identification and can alert security personnel to potential IEDs, firearms, drugs, and other illicit materials.
Tens of millions of vehicles cross U.S. borders every year, challenging Customs and Border Protection to carry out inspections without compromising standards. Given the scale, CBP officers have often had to choose between inspecting only a small percentage of vehicles at random or introducing unsustainable levels of friction. In response, the organization has adopted automated scanning solutions such as X-ray portals to help them maintain high standards of security while minimizing the duration of inspections.
One of the solutions that has drawn increased interest is under-vehicle inspection systems (UVIS), which can scan the undercarriage of passing vehicles and alert agents to potential threats in a matter of seconds. Those vehicles can then be subjected to a more intensive search.
While some vehicles crossing the border are repeat traffic, such as commuters, this is not a situation in which users can rely on a database of vehicles. Instead, they require a solution that is capable of detecting threats on the first pass, identifying anomalies that could signify a threat, even without previous scans being done.
First pass detection capabilities are the result of years of work in the field of deep learning, teaching the algorithms to understand what the parts of a vehicle are supposed to look like, even under less-than-pristine conditions.
While border inspections are one application for deep learning, there is a wide variety of potential uses for this technology across a range of verticals.
Over the next few years, deep learning is expected to have a bigger role in the security space, while unique identifiers and first-pass detection capabilities will likely become the standard for under-vehicle inspections, not only at borders but across the security industry.
Civilian use cases for this kind of technology could include deployment at access points, secure payments when a vehicle drives in or out of a commercial facility, smart city and traffic management, stolen vehicle sales prevention, and many more.
The software described above combined with the right camera and scanners can aid in the creation of new methods of verification and added security layers, and the principles behind inspecting the undercarriage of a vehicle can also be applied to other modes of transportation, such as airplanes, ships and cargo containers.
Leveraging AI to replace manual processes can make verification procedures more accurate and efficient, thus enhancing security. Computer vision can be used to view objects in different environments and, while categorizing and learning the images, can create valuable database entries, alerts and knowledge management.
About the author: Amir Hever (amir@uveye.com) is CEO of UVeye (www.uveye.com).