SIA Tech Brief: The Case for Sensor Fusion –Monitoring Spaces for Real-Time Change Detection and Immersive UX
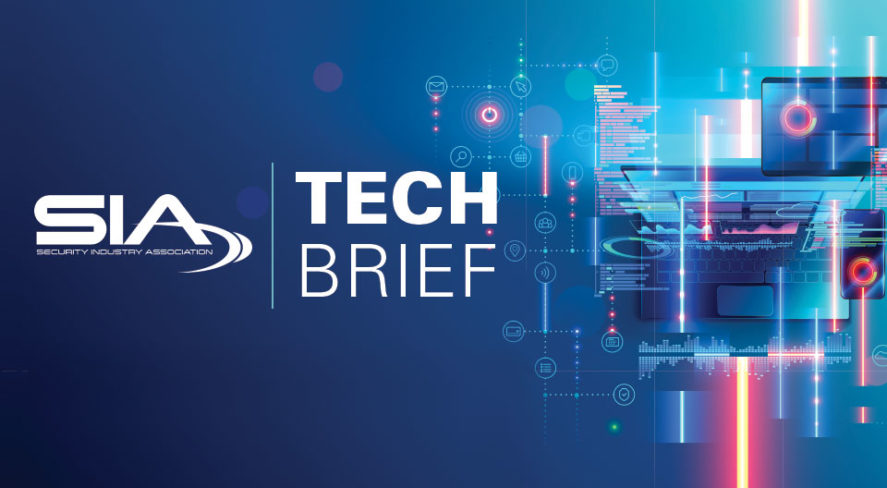
IP video is joined by thermal imaging, radar, LiDAR and 3D imaging to support better decisions by neural networks
Introduction
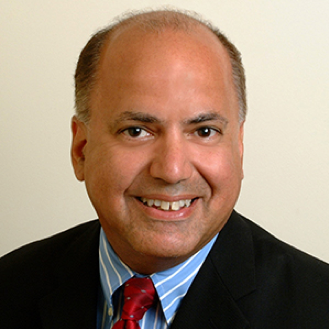
Sensor fusion is the ability to bring together inputs from multiple radar, LiDAR, thermal imaging, 3D imaging and visible light cameras into a single model. This could be images or video streams of the environment at a facility entrance or around a corporate campus, city, public space, critical infrastructure or transportation artery. The resulting model is more accurate because it leverages the strengths of the different sensors, more appropriate because it uses thermal imaging, LiDAR and 3D time-of-flight (ToF) sensors for object classification with anonymization.
In the Predator science fiction franchise, the extraterrestrial creature tracks, surveys and targets other species for sport. It sits silently in a tree with its prey unaware below. Its bio-mask increases its ability to see in a variety of spectra, ranging from low-infrared to high-ultraviolet, and also filters the ambient heat from the area, allowing it to see things with greater clarity and detail. Sensor fusion is similar in function: Spectra is selected to extend human vision for a deep neural network (DNN) or convolutional neural network (CNN) to make the best “guess” about who or what is in the scene, how long they’ve been there and whether a given “behavior” has been seen before.
CNNs are a class of DNN most often used for visual imagery, speech recognition and natural language processing (NLP). A CNN analyzes whether or not a given pixel is part of an object, such as a gun. If multiple guns of different types are in the same image, a regional CNN will virtually separate the objects believed to be guns, resize, zoom and analyze them individually. CNNs are widely used in AI systems-on-chip (SoC) and AI processors.
CNNs are also versatile enough for NLP, the ability to analyze, understand and generate human language. The next stage of NLP is natural language interaction, which allows humans to communicate with systems using normal, everyday language to perform tasks. Because our interactions can be via speech, keyword, keyboard, pointing device, game controller or, in the near future, gestures, the fusion of sensor renderings is known as user experience (UX).
Fusion of Sensor Data; ADAS Impact on Other Industries
Robotics, health care, information and communications technology, utilities, chemical, public safety, and security industries are delivering better services from this expanded vision through sensor fusion. Vehicles equipped with advanced driver assistance systems (ADAS) and autonomous vehicles (AV) use sensors like cameras, radar, LiDAR and ultrasonic to help the vehicle perceive its surroundings. This perception is critical and ensures that the AV can make the right decisions: remain in lane, reduce speed, stop, accelerate, turn. The AV market and the push for greater safety make 3D imaging, LiDAR, radar and thermal sensors affordable alternatives or enhancements to visible light imaging. Any combination of this wide range of sensors provides a visual “fusion” of data while preserving privacy and displaying a spectrum most closely suited to recognizing a potential threat. Facility security and public safety have already begun the transition to “alternative” visual sensors and processing using 3D imaging, radar, LiDAR and more.
One example is the Ambarella Computer Vision AI processor that renders detailed 3D images of people and vehicles in real time at significant cost savings. If privacy is required, the “camera” with these sensors provides another stream of detailed wireframe or point-cloud-renderings without the visible light imagery. In other words, the greatest detail of the person and what they are carrying, without facial imagery, thus preserving privacy.
These AI vision processors are already used in a wide variety of human and computer vision applications, including video security devices, ADAS, electronic mirrors and robotics. For example, the high-end Ring Doorbell Pro 2 delivers enhanced 1536p HD video with an expanded head-to-toe view, bird’s eye view with intruder motion history and dual-band Wi-Fi, and it operates on a low-power, high-performance Ambarella SoC.
LiDAR uses pulsed lasers to build a point cloud, which is then used to construct a 3D map or image. A ToF sensor in a 3D camera is able to reliably reconstruct individual objects in 3D in real time, with detail and with fast frame rates. The “depth maps” can be colorized or even merged with the RGB visible light camera.
Thermal imaging sensors, together with AI algorithms trained on weapons and improvised explosive device (IED) data can provide improved public safety, as recent years have demonstrated an increasing trend toward IED attacks that are suicide-initiated.
The case for extending human vision using multiple spectra with privacy in smart spaces, sustainable, safety and security systems enables DNNs and CNNs to achieve greater accuracy in object and behavior detection and recognition. The following use cases leverage sensor fusion.
Use Case: AI-Based Entry Screening
With COVID-19, entry screening now has three or more parts: one step to make sure you should be visiting the facility, another to verify your identity and yet another for a health check.
For a converged and optimized security team that recognizes the value of AI, this has been significantly optimized. If a visitor drives a vehicle, they can be screened by an AI-based automated number plate identification device incorporating visible and IR light detection and multi-core imaging processors and capturing vital data like vehicle make/model, the number of passengers, vehicle speed/behavior, vehicle tags and contraband/explosives detection. On entry into the facility, there is no longer a need for security staff to manually perform screening. At an entry portal, the visitor’s biometric factors may be verified, a scan for concealed weapons conducted and a health check performed using the subject’s basal temperature.
A concealed weapons detection system from Athena Security includes a walk-through metal detector leveraging AI processing from a suite of sensors, including magnetometer, induction, LiDAR, thermal and visual cameras to scan one person at a time walking at normal speed through unobtrusive pillars. At a maximum flow rate of 3,600 people per hour, it is 10 times faster than legacy metal detection and even faster when considering legacy secondary screening methods.
Use Case: Delivery via Vehicle; Vehicle Theft
Vehicles are often the targets of “smash and grab” crimes or sophisticated gray market parts distribution. A successful delivery of goods at a residence or business might involve some recognized behaviors. However, visual sensor fusion used with an edge AI computer vision perception SoC or AI processor can process unclassified behaviors in real time.
Use Case: Pre-Entry Behavior
When multiple people are in a video doorbell’s field of view, it can be useful to see what they were doing before pressing the doorbell, as well as their distances relative to the user at home or at a commercial entry. This is also known as AI distance inference.
Use Case: Complex Building Lobby
A building lobby of a multitenant facility might require screening, performed continuously and accurately. In this case, each of four 4K visible-light RGB cameras were paired with 3D imagers producing eight streams, four of which used CNNs to process skeletal poses, object detection, face detection and something the person has with them (a body part). These applications enable quick alerts on slip-and-falls, crowding, package theft, mask wearing and weapons.
Use Case: Face Matching Access Control
Legacy face matching algorithms can often be spoofed by 2D images of the person on file. When a 3D ToF camera is used together with an RGB camera and SoC capable of fusing both streams and images via CNNs, false positives are statistically eliminated and trusted personnel entry is achieved.
Use Case: Anonymous Occupancy Sensing
Detailed visual imaging is not always necessary to maintain safe occupancy in a building or space. Thermal imaging, 3D imaging, radar or LiDAR streams can be processed by neural networks and an accurate occupancy level for a given spaces can be determined in real time, or even projected by time, while maintaining privacy. At an approximate cost of one-third of a fixed IP camera, a smart building multisensor includes infrared, acoustic, temperature, humidity, near-field communication and Wi-Fi for guard tour, HVAC control and catastrophic equipment failure detection. The primary purpose is occupancy sensing, but the device can check how long people have been in one place, without moving, while maintaining their privacy.
Sensor Fusion Expansion
In health care, patient outcomes and standardization of care continue to benefit from smarter and more connected devices, with their data fused to not only identify the most notable diagnoses in a given region, but also a life-saving differential diagnosis that might not have been considered. Greater perception leads to better identification of potential threats, in both health and security.