Context is Key – For Both People and Artificial Intelligence
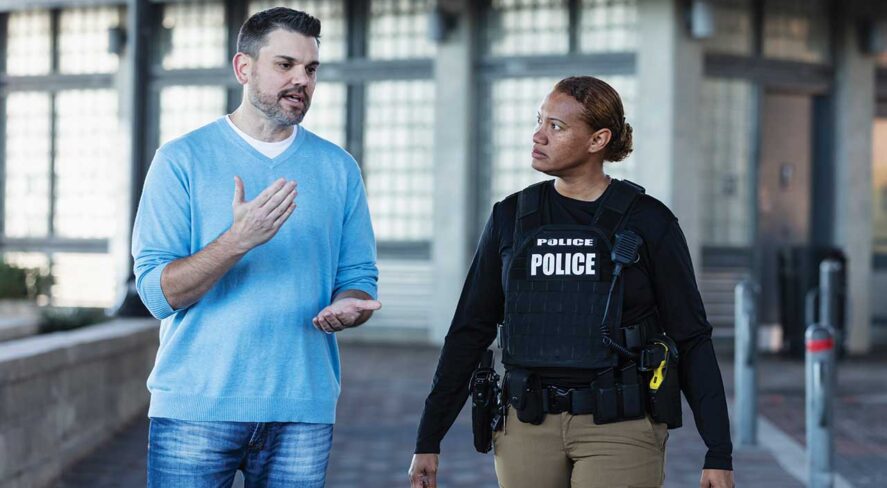
Multimodal systems can make the leap from identifying to understanding
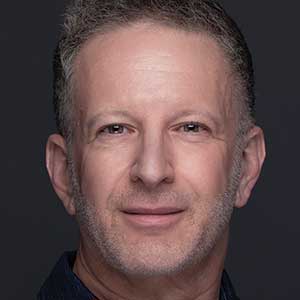
A group of people rushes into a store and coordinates to quickly steal a large amount of high-value merchandise.
A belligerent hotel guest confronts and threatens a front desk clerk working alone late at night.
A security officer at a large office park finds an intoxicated, mentally ill trespasser wandering around a parking garage.
A frantic parent comes to a shopping mall security office to report their young child is lost.
Whatever the differences among these varied security incidents, each of them at its core is a social interaction.
The intelligent deployment of security resources centers on understanding and anticipating the complex human behaviors that lead to everything from retail theft to active assailant attacks. In fact, the entire security ecosystem can be seen as a vast network of social interactions. Better understanding and management of these interactions translates into more effective, efficient security.
Ideally, of course, security incidents should be prevented – or at least detected – before they become serious. Prevention and detection are also inherently social processes, because they require security organizations to have deep knowledge of how people behave and interact in ways that can lead to disorder, crime and violence.
Given the inherently social nature of security incidents and their prevention and resolution, the success of the security industry requires optimal leveraging of the exponentially growing amount of social data at its disposal. Artificial intelligence (AI) technology can transform the way security organizations analyze and use the massive amount of social data collected by video and audio devices, including fixed cameras, body-worn cameras and mobile cameras deployed on vehicles, phones, drones and other platforms.
At present, the ability of the security industry to analyze video-based social data is fairly basic. Most current video analytics technology is limited to the simple detection of people in a given location without a deeper understanding of what they are actually doing. At a more advanced level, there are newer computer vision technologies that can recognize emotions and facial expressions or identify individuals. However, despite their powerful capabilities, these tools are largely incapable of analyzing the back-and-forth dynamics of human interactions and detecting essential social processes such as conflict, cooperation, de-escalation, violence, or the use of force. Given these limitations, the security industry is left in the operationally and financially untenable situation of collecting massive amounts of high-value video and audio social data that it cannot put to full use.
Video cameras are the security industry’s single largest source of data about the myriad human interactions that occur before, during and after security incidents. In addition to capturing anomalies and serious security events, video data also contain invaluable information about the normal conditions in which nothing goes wrong. Understanding these baseline conditions at scale is one of the most important steps toward the enhanced detection and prevention of dangerous aberrations.
Video surveillance networks around the world record thousands of petabytes of data every day. However, most of this data is never analyzed, let alone applied in ways that could optimize both operational success and commercial value. As the security industry evolves to meet urgent challenges posed by mutually reinforcing factors, such as the steep rise in property crime and general public disorder and the parallel contraction of government policing services, it is more important than ever for the private sector to think strategically about how to optimize the efficient use of social data in security environments where law enforcement resources are increasingly unavailable.
A recent SIA Technology Insights article by Matt Powell stated that AI-driven video analytics are creating a “third wave” in surveillance innovation with the potential to “pay dividends as a force multiplier for end users and a moneymaker for integrators.” Recent developments and emerging trends in multimodal AI and the related field of computational social science offer new opportunities for the security industry to enhance its capabilities, efficiency and competitiveness by leveraging social data.
AI uses computer technology to approximate human abilities such as visual perception and language understanding. AI also enables the analysis of vast amounts of data that are too large and complicated for humans to manage without powerful computational resources. Computational social science refers to the use of computer tools like AI in conjunction with research on human behavior to address complex problems in areas ranging from crime to public safety to health care to poverty. When the latest AI technology is combined with social science, the practical benefits can be huge.
The three kinds of AI most important for analyzing social interactions in security environments are computer vision, natural language processing (NLP) and speech processing. Computer vision uses AI to automatically identify patterns of behavior, movement and emotion. Computer vision tools can also detect various kinds of events such as violence or a medical emergency. NLP uses AI to understand the content of speech – what people are saying – while speech processing uses AI to analyze the quality of speech – that is, how people are speaking to each other (tone, pitch, etc.). NLP and speech processing are especially important for the analysis of data collected by video devices like body-worn cameras and access control cameras that also have audio recording capability.
Multimodal AI refers to systems that integrate computer vision, NLP and speech processing to create analytic capabilities that approximate what humans do in live social interactions. When people interact, they simultaneously analyze each other’s behavior, speech content (what is being said) and speech quality (how words are spoken). This is true for both face-to-face encounters and virtual interactions such as video meetings. For example, it is easy to identify which people in an online meeting are actually listening and participating and which ones are “checked out” or doing other work.
People also intuitively understand the unique context of various interactions. Trying to get to a seat on a crowded airplane, checking out at the grocery store, or having a family dinner. This context provides us with the “metadata” we need to help make sense of all the other information we gather from observing people. Plainly said, other people’s behavior and language only makes sense to us because we understand the context in which it is occurring.
Multimodal AI functions like a human observer and analyzes vast amounts of social data collected by surveillance systems. By integrating social data analytics with wider metadata, security organizations can radically enhance the efficiency and safety of their operations. This process of integration requires drawing on computational social science as well as domain expertise.
Simply having the latest multimodal AI technology is not enough to effectively analyze vast amounts of security-related social data. The analytics provider must also understand general principles of human behavior and how those principles function in each unique security environment. While AI will never replace human judgment as the heart of effective, ethical security, it can dramatically enhance the use of social data in beneficial ways that uphold the safety, welfare and dignity of all people.
Perhaps the most important feature of systems that combine multimodal AI, computational social science and domain expertise is their capacity to understand the dynamics of entire social interactions, rather than just the isolated behavior of individuals. Multimodal AI can examine myriad interactions between members of the public, employees, security personnel and others to generate fine-grained understandings of what causes, prevents and mitigates a wide range of security incidents. This kind of understanding not only helps to improve the efficient deployment of security personnel and technology, it can also inform best practices that can enhance safety and reduce liability.
Like any powerful new technology, multimodal AI has vast upside potential while also raising a host of complex legal, ethical and privacy questions. Answering these questions cannot be reduced to conference room abstractions, it can only be accomplished in the real-world context of disciplined, gradational testing, piloting and operational implementation.
The proverbial AI “cat” is out of the bag. The question is no longer whether it is possible to analyze the vast flow of social data collected by security video and audio networks, but, rather, how to do so in a transparent, rigorous manner that addresses the legitimate needs and concerns of diverse stakeholders.
The security industry can further this process and ensure its success and integrity by asking the right, tough questions. How should security-related social data be structured, searched and analyzed? What should be the highest priorities for the analysis of security-related social data? How can accuracy be increased, error rates decreased, and biases mitigated? What are the legal, business and ethical implications of various data analysis strategies? What safeguards are necessary to ensure that social data analytics are ethical and defensible?
Finally, what are the risks of affirmatively foregoing the opportunity to analyze security-related social data as its powerful potential to improve public safety becomes increasingly evident?