Transforming Data into Actionable Intelligence
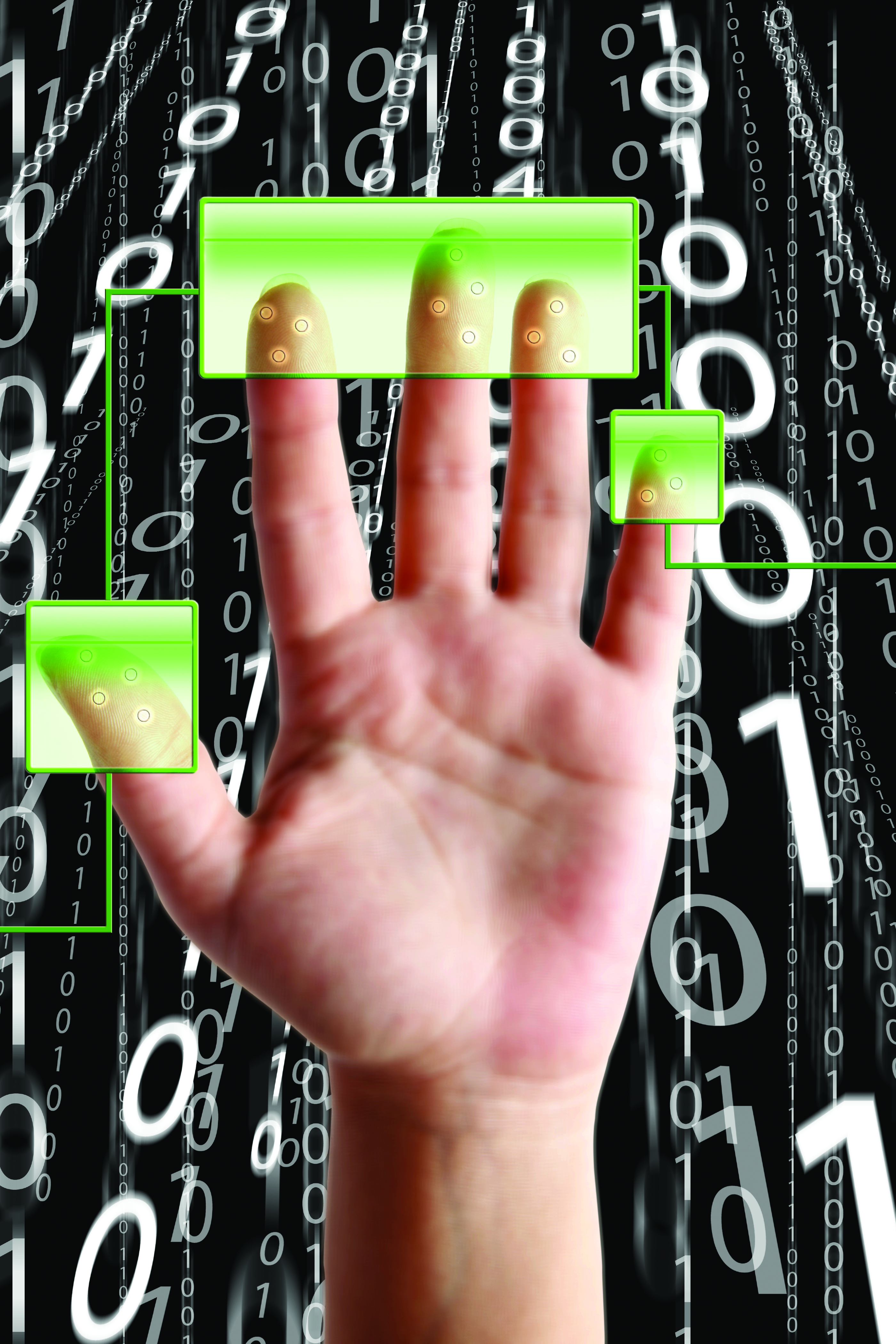
New solutions can identify insider threats before it is
too late
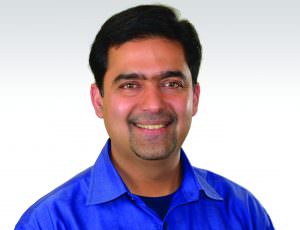
Ajay Jain, Quantum Secure
In every industry, data drives decisions. This might be as simple as an organization reviewing financials to determine whether an expansion is feasible, or it could come in the form of more complex analysis, such as examining a pool of information to identify which customers might be about to defect and determine how best to retain them. Whatever form analytics may take, organizations increasingly recognize their potential to transform raw data into actionable intelligence.
With data analytics, it stands to reason that more data should equate to more insights that enable the most informed decisions and the best outcomes. And there is a growing abundance of data that is generated by and available from an expanding number of disparate sources. Access control, video surveillance, human resources, traffic control, point-of-sale and many other systems and devices all contribute to the flood of information. Unfortunately, many organizations have still not found an efficient way to deliver on the promise of “big data.”
Physical identity and access management (PIAM) solutions utilize the new science of predictive analysis to help transform the security department into a proactive strategic partner that plays an integral role in an organization’s business and growth. While conventional security solutions such as alarms and video surveillance systems provide comprehensive real-time coverage and alerts, they are typically considered to be reactive rather than proactive resources. Worse yet, in some situations, the security system’s own shortcomings can render it ineffective. For example, because more than 95 percent of alarm activations turn out to be false, security officers may begin responding more slowly, or not at all, to the alarm. In essence, the alarm monitoring process itself has inadvertently trained people not to pay attention to alerts when they occur. As a result, threats may go undetected – or by the time it becomes evident that there is a real incident in progress, it may be too late to do anything about it.
PIAM systems with predictive capability, on the other hand, are designed to analyze a wide variety of data from multiple systems and devices to identify statistical patterns and trends. This analysis helps identify predictors for future incidents, known as indicators of compromise (IOCs), which may include changes in access or behavioral patterns, such as entering a facility at unusual hours or locations, or attempting to access unauthorized areas. This analysis allows organizations to identify potential threats or business opportunities early on. An added benefit is that these predictive analysis systems are capable of learning and improving over time, and are often able to identify patterns that were never expected or that most likely would not have been uncovered without that level of processing and automation.
This use of metrics is a significant element of predictive analysis, allowing PIAM solutions to create a picture of what normal looks like and, from there, extract useful information out of the mountains of data. As an example, to determine the effectiveness of security and operational policies, it might be necessary to know the number of visitors who enter a facility during specific time periods, the time it takes to process those visitors, and how that affects start-times for employees.
When these metrics are combined, lobby staffing levels can be understood and managed more effectively. Or, in terms of operational effectiveness, the duration of the process for new hires to receive access approval can be used to find areas where automation may have the highest return on investment.
Metrics are also proving to be vital in the fight against insider threats, which are increasingly prevalent security concerns for organizations.
Given the complex psychology involved, insider threats can be incredibly difficult to understand. However, using predictive analysis, one can develop an idea of who might be most likely to attempt an attack from the inside.
First, a profile can be created based on each person’s current access, time of employment and time since his or her last access audit, background check or other mitigation control. The access profile is the first element that indicates each person’s inherent risk to the organization.
A triggering event, such as a bad performance review, a missed promotion or something similar is another indicator that might precede an insider breach. While this sensitive data must be properly managed and handled, it could potentially serve as a second predictive element. Information related to these events is securely stored in the human resources system and could be used by authorized individuals to generate an initial red flag that an individual might pose a rising threat.
The third element is behavioral information. This includes data such as which individuals might feel they have reason to take some kind of criminal action and have the physical access needed to do it, and who among these individuals also has a history of activity that might indicate an imminent threat.
Behavioral information can be analyzed in many ways. For instance, you may want to see a report showing every time a person entered the premises and every door he or she accessed to help establish that person’s normal routine.
Each element of the insider threat risk profile provides a different perspective and builds a larger picture that can be used to reduce the danger or improve overall understanding. Access audits can be focused on individuals with high scores in multiple elements, including high levels of access, and used to remove unneeded access. Reactive alarm management can even be given new meaning. Sharing the behavioral change and risk score information with the security operations center allows them to react with real understanding instead of being forced to treat every alert the same way.
By mining the accumulated data with established metrics, security can analyze information to look at patterns across a large number of employees over a long time period to identify things that may not be obvious or intuitive.
These patterns can then be used to develop additional metrics to indicate a potential threat. If an employee exhibits not only differentiated behavioral patterns but access patterns as well, those IOCs show that he or she is a higher risk and, as such, should be subjected to additional scrutiny. For those employees who have been flagged in the system, future deviations from their routines, such as coming into or leaving work at an unusual hour or accessing areas of the building or information systems they have never accessed before, will generate additional red flags or even alarms, but now the alarms will have context provided by predictive analysis.
One real-world example of the effectiveness of predictive analysis can be found in a company that was experiencing the loss of equipment over a period of time. At first, company officials were unsure who was behind the thefts, but they thought it might be the work of an insider. One factor was that the losses were mostly being reported in the morning, which would indicate that the thefts were likely occurring after hours.
Based on this initial information, the company began to analyze data to examine employee activity, beginning with identifying any employees who were behaving outside of their normal routine. They were able to determine those routines using data that had been collected from a number of systems, including access control. This analysis led them to discover a particular employee who had started to access areas and facilities he was authorized to enter but had never previously entered. They were also able to determine that this access was regularly occurring outside of the employee’s typical hours, often in the late evening. A final factor was that these abnormal behaviors correlated closely with buildings where the equipment was disappearing. From there, the company set an alarm for any time he accessed a new area late at night, even though he was authorized to enter the area. The next time he did so, an alarm was triggered. When security staff responded, they caught the employee in the act of disassembling and preparing to steal yet another piece of equipment.
Without predictive analysis technology, the challenges related to data collection and investigation are daunting. Most organizations have no problem identifying what they hope to learn from data analysis, but they lack the capability to collect and organize all of the data relevant to that goal. Without a comprehensive means of collecting and organizing large amounts of data – not to mention analyzing the data for insights that will help them make smarter decisions – organizations end up with business as usual.
Post-incident analysis of security breaches has repeatedly uncovered the fact that there had been enough relevant data stored in disparate sources for predictive analysis to have identified a potential breach, alerted staff and provided actionable intelligence that could have prevented the incident from occurring.
While the idea of building an in-house custom application for predictive analysis may seem attractive, the requirements for big data analysis are often not well understood by in-house developers and business owners. Further, a professional subject matter expert can provide a better view of how to integrate company processes, business challenges and unique needs into a solution that can be developed more accurately and implemented less expensively than it could from within the organization. All of these factors underscore how important it is for organizations to seek outside assistance when considering a predictive analysis model.
Although technical at its core, predictive analysis must be integrated as a security activity, and subject matter experts who have experience in both security systems and data analytics can realize this goal for an organization. A subject matter expert can also help to ensure successful implementation and integration of predictive analysis so that organizations can benefit from the full potential of these powerful solutions. They work closely with the organization to identify and integrate all of the data-generating systems and devices – not only to ensure that data is properly collected, but also to ensure that it is high quality and will contribute to the effectiveness of the predictive analysis solution. The outstanding value this process will ultimately deliver will be evident in the capability of the organization to develop deep insights that lead to more efficient operational decisions and that reduce its risk profile.
As the proliferation of data continues, subject matter experts become even more necessary to organizations as they can bridge the gap between technical understanding and practical use. This ensures that identified trends or predictors are actionable and have an impact on security and business operations.
The actionable intelligence delivered by PIAM solutions with predictive analysis technology allows organizations to identify potential threats and opportunities in real time and apply proactive measures to guard against breaches or reap the benefits of unanticipated changes in business practices or policies.
Lacking the means to properly collect and analyze data, or not recognizing the opportunity for change until it is too late to be impactful, is clearly detrimental to security operations. Working with a subject matter expert to harness the full power of predictive analysis allows organizations to transform their big data into intelligence that drives more effective and efficient security practices and that creates competitive advantages for their businesses.
Ajay Jain (ajain@quantumsecure.com) is president and CEO of Quantum Secure (www.quantumsecure.com).