Reducing Retail Shrink with Business Intelligence Software
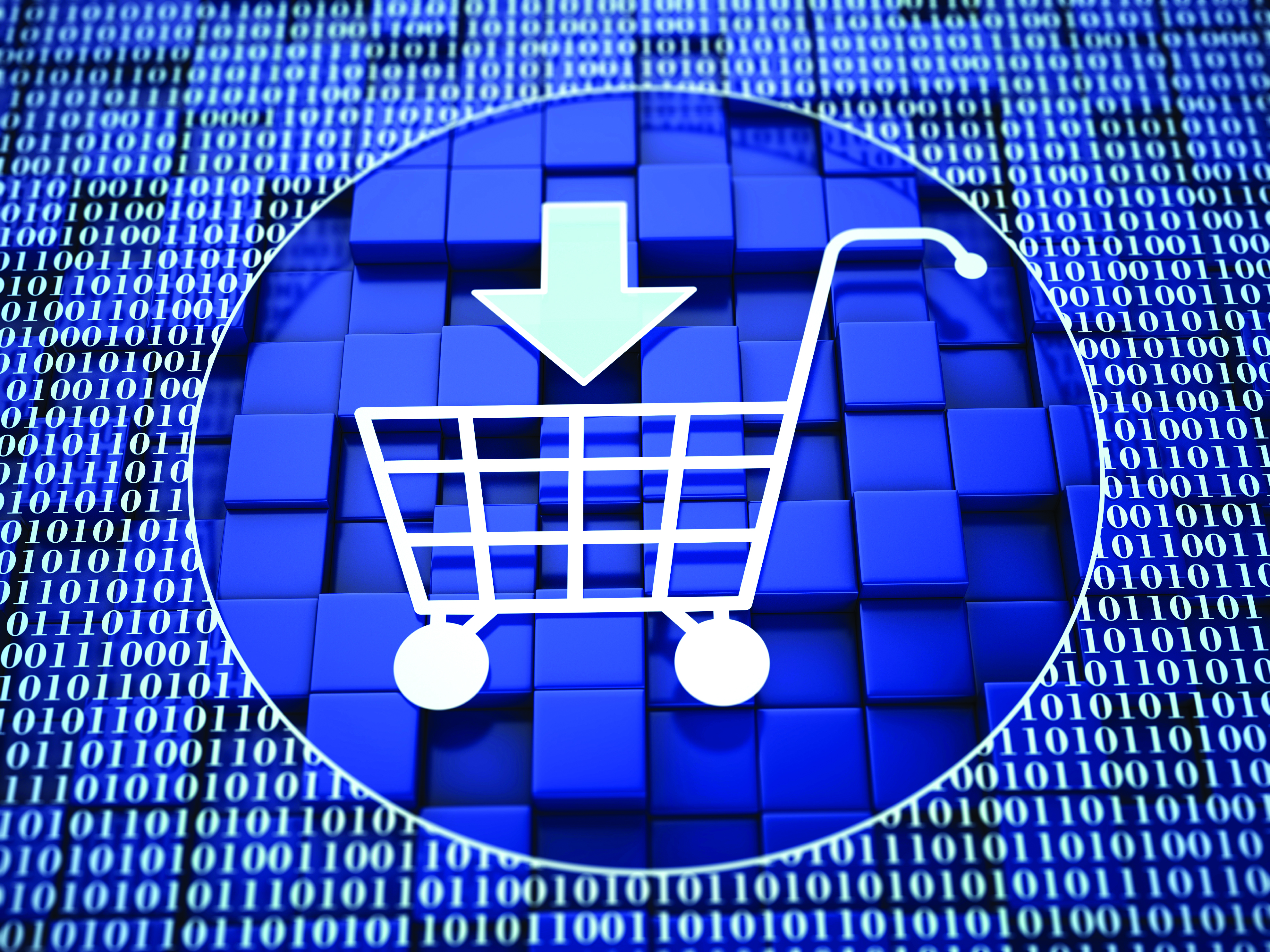
Data mining can be a valuable new tool for loss prevention professionals
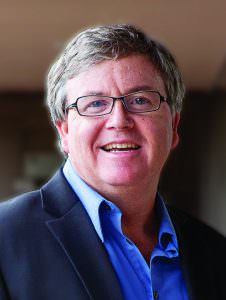
Charlie Erickson, 3xLOGIC
Retailers, like most businesses, are attempting to do more with less in an effort to increase competitiveness and profitability. Hardest hit have been corporate support organizations (Target and Best Buy are recent high-profile examples), where corporate loss prevention (LP) personnel who specialize in identifying and investigating corporate shrink typically reside. These developments, combined with shrink numbers that exceeded $128 billion in 2014, are exacerbated by decreasing employee loyalty resulting from the fact that many retailers have reduced employees’ weekly hours. This has led to some employers increasing the number of their part-time employees by 30 percent or more. In fact, the Bureau of Labor Statistics estimates that the number of involuntary part-time workers nearly doubled from 2006 to 2013, and that trend seems to be continuing.
Trying to Do More with Fewer People
Retail employees now frequently have to juggle multiple jobs to earn the same income, further driving down already low employee loyalty. This has created a perfect storm: fewer people to oversee a greater number of workers who have relatively low-wage jobs and less than stellar loyalty to their employer. Is it any wonder that upwards of 50 percent of retail shrink is internal? Employees stealing from their company – the type of theft that is not opportunistic like most shoplifting – is chronic.
The focus of retail loss prevention is on return on investment (ROI), to be sure, but, just as important, these tools must demonstrate a return on time invested (ROTI), as well. That is, if a person is going to spend an hour using a given tool, it must be more productive than an hour using other approaches and tools. Also, the better, newer tools must be able to capture and apply the insights of LP specialists and then make the results available to a wider audience within the company. Some retailers outsource this function to firms whose employees have little insight into the company itself. This means the best LP tools have to be intuitive and easy enough for just about anyone to use.
Employee theft that originates at the register is a huge and persistent problem, generally making up nearly 50 percent of the loss in any retail operation, and some retailers contend theft by employees accounts for as much as 80 percent of their total loss.
This means that, day in and day out, a single employee can steal from an employer using the same methods, if undetected, and losses exceeding thousands of dollars are not uncommon.
Therefore, catching a single dishonest employee generally has a greater impact on shrink than catching several shoplifters who may only steal opportunistically once or twice from that retailer.
The sooner a company can identify and remove thieving employees, the greater the impact on reducing shrink. An LP manager recently talked about an employee who was doing item returns for cash and started out stealing $8 on the first night. By the end of a month, when the employee was finally caught, he was taking more than $600 a day, for a total loss of $18,000. The employee is now in jail, but the retailer is out the $18,000. Think about a company that has 1,500 stores across the country. Now, imagine only 1 percent of the stores have dishonest employees like Mr. $18,000. Such a scenario could cost the retailer $270,000 in just one month.
Implementing Business Intelligence Analysis
While a number of companies have specialized for some time in tools to help identify suspect transactions, the focus now needs to be not only on how to identify a suspect individual transaction but also on how to deploy a comprehensive, data-driven method to identify employees who are the worst offenders, by company, region, division and store. All retailers have learned over time which type of point-of-sale (POS) transactions or combinations of transactions may indicate employee theft. Typically, retailers will have 20-30 types of transactions, and of these 20-30 types, some are much more likely than others to be theft.
A great way to find top offenders is to assign a weight to each type of transaction or exception (giving higher weights to transactions with higher potential for theft), so that chronic offenders visibly bubble to the top of the list for each transaction (or exception) monitored. Doing this in combination with a straightforward and quick review of all transactions and the associated video increases the effectiveness of LP professionals and also provides a tool that regional managers and others can use to get involved and contribute to reducing shrink. This sounds great, but what is happening in the actual stores?
A recent Aberdeen Group study indicated that 45 percent of retailers struggle to get timely data and only 26 percent have implemented any exception reporting program. Since management tools that deliver timely data are increasingly available, and there is minimal capital investment to implement a cloud-based “big data” exception reporting solution, the percentage of retailers who implement such reporting systems is expected to increase significantly over the next few years.
Business Intelligence in Action: Three Mini Retail Case Studies
Simple to Use
One company implemented a POS monitoring program for its 1,500 stores and had 8,000 employees processing more than 2.5 million transactions every day. A webinar training session was scheduled to get the LP team started on the software, and the email invitation included a login and password that the head of loss prevention could use to access the solution’s dashboard. Five minutes after receiving the email, he replied to the invitation, “I love it, it’s so easy to use, I just caught the first person!” The best, most effective business intelligence packages must be very easy to use and capable of finding “the needle in the haystack” without complicated maneuvers and user-written code. It must provide that all-important ROTI.
This company now projects that one of its analysts will reduce shrink by approximately $1.2 million in one year using the software package.
Integrated Video for Instant Review
The inability to quickly review video associated with POS exceptions has traditionally been a weakness in exception-based reporting systems. Typically, systems provide the time and date of the POS exception and then the end user has to crank up the DVR software to manually input the parameters to be applied to request the relevant video. In such scenarios, the end user has to wait for the video to be uploaded from a distant store. In most retail applications, the network links to the stores are very low speed, and security video almost always is allocated only a portion of the network connection, so more critical store operations such as POS and credit card data can be processed. As a result, it can take more than 10 minutes to review a one-minute POS transaction. This is not very good when measuring ROTI, especially when it is repeated over and over.
The best business intelligence software utilizes a scheme in which videos of all POS exceptions that show a high probability of theft, and therefore are expected to be viewed, are uploaded to the cloud in the background, when bandwidth is available, where they can then be played in a YouTube-like format. By using such a video preload capability, one end user’s corporate investigators, who had been closing just one or two cases a month, closed 100 cases in the first full year of use. Because they could review video quickly, they were able to look at far more exceptions and close more than five times the cases on an annual basis.
Using Video for What Can and Cannot Be Seen
Tight integration with video enables LP professionals to create compound exceptions that are based not only on POS transaction data but also on who is in the camera scene. Using that capability, an exception can be created for a product return when no customer is present. Video analytics are used to determine if a customer is in the area where a customer should be standing during a return. If there is no customer, then the exception is flagged. The odds that this type of transaction involves theft are very high. When this function was enabled for one company that had 100 stores, they caught 30 employees in the first month. Several were trusted employees in good standing who had worked for the company for more than five years, proving you often really do not know where your shrink is coming from.
What’s Next in Battling Shrink?
One of the benefits of using big data over the last couple of years is that the cost of creating data sets with billions of transactions is low, and adding the ability to search those transactions at an equally low cost is creating new opportunities to more effectively mine that data.
It was customary to keep a few months of transactions per retail location, with searches possible for only one location at a time. Now, several years of transactions can be saved, and searches can be quickly performed across the enterprise.
With this much richer data set, it is possible to study employees who have been caught stealing and compare them to current employees to help spot bad behavior quickly so it can be stopped with a conversation and disciplinary action not requiring termination.
This prevents significant loss, which is the key objective of any LP specialist. This may also “save” an employee from being terminated and getting a record that will negatively affect his or her entire career, while, at the same time, saving the retailer the cost of hiring and training a replacement.
These data sets can also be used to monitor new employee performance, determine what type of training is the most effective, and identify when additional training is required. In addition, it can highlight patterns of activity where stores or regions are not conducting business according to corporate guidelines. For example, it can determine which employees are checking IDs for tobacco and alcohol purchases, rather than simply doing a date override because the customer “looks old enough.” Customer demographics are consistent over time at the same location, so checking IDs should be equally consistent for all employees.
Retailers have many other rich data sets that fit nicely into an advanced business intelligence software package. Data from alarm panels, for example, can provide many insights. Simply by looking at opening and closing times, headquarters operations staff can quickly see which stores chronically open late or close early, and locations with higher-than-average numbers of unscheduled openings can be investigated. Monitoring phone records, meanwhile, can point to stores where employees may be spending excessive amounts of time on non-work related activity. The list goes on of data sources that may seem mundane but that provide valuable insights into behavior that is potentially hurting productivity and reducing profits.
Retailers have only started to reap the benefits from mining all the data they are collecting across tens of thousands of locations, and the ROI – and ROTI – will only increase as more data sets are added. When end users start asking questions about their data that would have been impossible to answer just a few years ago, they will come to understand new insights that they only dreamed of, and their wish lists for tools to further reduce shrink will really start to grow.
Charlie Erickson (charlie@3xlogic.com) is vice president, product management, at 3xLOGIC (www.3xlogic.com).