The State of Security Video Analytics
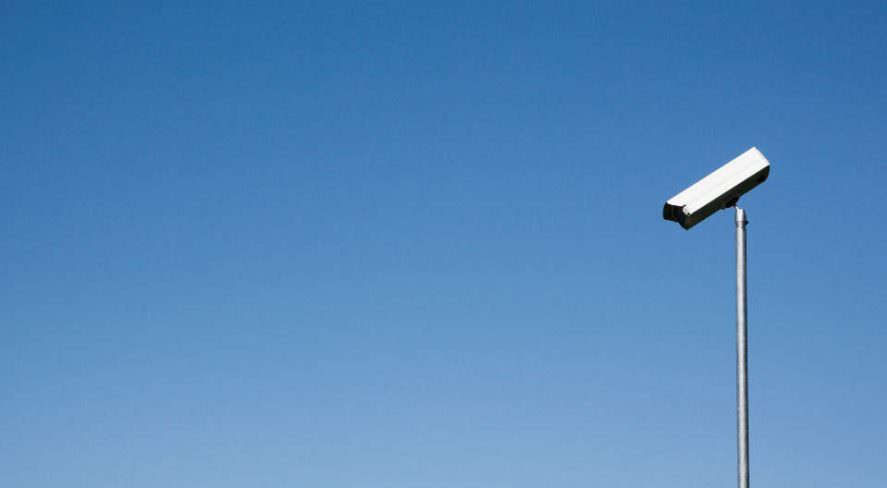
In recent years there has been an appreciable increase in video analytics research and development, and as a result, the video analytics capabilities for security video surveillance systems have been advancing significantly. As with any rapidly advancing technology, consideration must be given not only to where the technology is at the current moment in time, but also to where it is going in the near future. This paper examines the current state of security video analytics technology, the nature of video analytics advances and the information technology trends that are enabling them. It also presents key considerations that apply to planning a modern-day video analytics deployment.
For decades, security video analytics has been a promising area of emerging technology. Although realization of that promise has been long in coming, accelerating advancements in computer vision — which is the parent domain of video analytics — are making up for lost time. Video analytics products are becoming truly useful in their target domains, with self-parking cars and virtual reality gaming being just two of many examples of the commercialization of video analytics applications. Serving as a “special set of eyes” on an organization’s facilities and events, security video surveillance technology is now capable of providing security insights and meaningful business intelligence, thanks to a new generation of arriving video analytics capabilities.
Download Available
The State of Security Video Analytics
By Ray Bernard, PSP, CHS-III
Emerging Technology
Video analytics is an area of technology that is focused on automating challenging security tasks such as: monitoring high numbers of live video streams; providing timely and actionable alerts for a wide variety of actions, activities and conditions; verifying alarms in remote locations; quickly searching large repositories of recorded video for content of a specific nature; identifying individuals, for example, by facial and gait recognition; and identifying anomalous behavior or activity of a concerning nature. In addition to the security and safety applications, video analytics is also a useful tool for business intelligence including, for example, in-store customer behavior analysis.
For purposes of this paper, the phrase “security video analytics” includes any video analytics applications that an organization may include in its security video surveillance system deployment, whether for security value, business operations value or both. As this paper was being written, a prominent security services company announced its new subscription-based services offering of security patrol robots. The data gathered by security video analytics stands today as an area of leading-edge technology with current capabilities offering much higher value than the previous generations of video analytics.
Video Analytics Progress
The basic definition of video analytics, also known as video content analysis or VCA, is the extraction of meaningful and relevant information from digital video. 1 It is the capability of automatically analyzing video to detect objects, characterize their appearance and movement, identify individual objects, and recognize their patterns of activity. Video analytics technology has broad application beyond the security industry, including in the following industries: 3-D gaming, animal behavior research, automotive and railway transportation, entertainment, health care, horse racing, industrial safety, manufacturing, mobile policing, retail, robotics, sports, traffic enforcement, and wildlife management. The broad range of applications provides good incentive for global investment in video analytics research and development (R&D), many aspects of which will benefit security video analytics.
Advances in video analytics technology are made possible by advances in the underlying information technologies, such as computer processing power, memory speed, parallel processing, solid state and hard drive data storage, high-speed databases, high-capacity networking, and artificial intelligence (AI). Video analytics is actually a subfield of AI. Video analytics capabilities fit both of the definitions of AI below.
The capacity of a computer to perform operations analogous to learning and decision making in humans, as by … a program for the perception and recognition of shapes in computer vision systems.2
The ability of a computer or other machine to perform actions thought to require intelligence.3
The ever-accelerating pace of advancement for the underlying technologies provides the research and development (R&D) efforts in video analytics with ever more powerful tools with which to develop and refine video analytics applications.
Two important video analytics improvement trends include higher detection rates and lower false alarm rates. In the U.S. National Institute of Standards and Technology’s (NIST) assessment of biometric face recognition in still images, the error rate halves every two years. In 2010, the best face recognition method matched 92 percent of mug shots to one out of 1.6 million images.4 Since then, the video analytics technologies have continued to advance in quality and effectiveness.
In 2013, the world’s largest supplier of automotive components (which also provides 90 percent of Google’s autonomous driving software) announced that it had begun sharing video analytics technology with one of its sister companies, a security systems manufacturing company. This is a noteworthy example of how the security industry will continue to benefit from analytics advances in other industries, some of which — like the automotive industry — have R&D initiatives that are significantly better-funded and better-staffed than R&D initiatives within the security industry.
Overall, four key factors are driving the ever-accelerating advancement of security video analytics:
- Information Technology Advancement. Across-the-board advancements in the information technologies with which video analytics offerings are built.
- R&D Investment. For many industries, there is growing investment in video analytics R&D initiatives, with hundreds of notable universities and research institutes around the globe participating in the efforts.
- Increasing Risks. The rising levels of homeland security and critical infrastructure risk — and the size of the physical landscapes that require effective video surveillance — heighten the need for more effective and more capable video analytics.
- High Security Industry Adoption Rate. Video analytics features are becoming commonplace in consumer- and commercial-grade security video products, and are demonstrating high value compared to earlier generations of video analytics technology.
These are among the many reasons to gain a clear understanding of where video analytics technology is today, where it will be tomorrow, and how to evaluate analytics companies and their technologies so as to select the capabilities that best fit a specific organization’s security and business needs. Today, there are many more considerations involved in video analytics selection than with the previous generation technology, due to the nature of video analytics evolution.
How Video Analytics Has Evolved
It is worth taking the time to understand the evolution of security video analytics, which requires examining the following aspects of the technology:
- Stages of security video analytics architecture
- Metadata advancement
- Accelerating trend in error rate reduction
Stages of Security Video Analytics Architecture
Video surveillance systems started out as fully analog systems known as Closed Circuit TV (CCTV) systems. In these early systems, one camera connected to one video monitor. There was no recording. Gradually, surveillance systems evolved into the fully digital, computer-based, networked systems that we know today — systems that are based on advancing information technology.
Video analytics functionality is provided by software. Software runs on a computer or a device with computer circuitry in it. Thus, security video analytics software runs on the computers and devices of a video surveillance system. As the computer and networking technologies of surveillance systems evolve, so does the design of video analytics software. However, the design of security video analytics software does not evolve just to keep up with changes in computers and devices, it evolves because of the need to improve surveillance capabilities. To date, video security analytics have been evolving to take maximum advantage of the computing capabilities of security computers and devices. However, the high value of video analytics is now beginning to influence the design of devices that run security video analytics, including cameras.
Physical Architectures
It is helpful to view security video analytics advances as having evolved into five physical architectures. The word architecture means “the carefully designed structure of something.”5 This paper uses the term physical architecture to refer to the physical device or computer where the analytics processing takes place. The first four architectures listed below, began with the previous generation of security video analytics, where the analytics processing was performed completely within a single device or computer. The hybrid architecture is a recent arrival, and refers to the system design in which some parts of the analytics processing are performed on one device, and other parts are performed on one or more different devices. This is a significant technological advancement, and will be examined in detail.
One physical architecture does not necessarily replace another, and all architectures have continued evolving to a greater or lesser degree over time. The order in which they are listed below is the order in which the architectures have come into use. They are:
- Appliance-based
- Server-based
- Camera-based
- Cloud-based
- Hybrid
Appliance-Based Analytics
Back in the time when digital video recorders (DVRs) were introduced for video surveillance, the DVR machines were not capable of running video analytics software. They didn’t have enough processing power or memory to do so. Thus, video analytics software had to be run on a separate and usually purpose-built device. An example is a video analytics appliance that automatically controls pan-tilt-zoom (PTZ) cameras to follow moving people and vehicles. The appliance runs computations on the video feeds from up to four cameras, identifies moving people and vehicles, and then issues control commands to a PTZ camera to zoom in and follow the target people and/or vehicles. The analytics appliance also provides a video monitor signal for displaying the video from the cameras that includes a rectangle outlining the person or vehicle being followed by the PTZ camera. There is no recording of any analytics information; the analytics data is utilized only in real time. The architecture for this appliance is shown in Figure 1.
Figure 1. Example of appliance-based analytics architecture
Appliance-Based Architecture
The appliance also outputs the original video camera signals for input into a DVR. Versions of these appliances are available today that encode the analog camera signals for network transmission to a network video recorder (NVR) or video server.
Due to the computing power required for the analytics computations and commands for real time PTZ control, for many years the analytics performance of such appliances was superior to that of video recording servers running similar analytics. Servers just couldn’t handle their normal video server functions and at the same time handle the video analytics computations as well.
Network Cameras and Encoders
One of the impacts of the arrival of network cameras and network encoders for analog cameras, was the elimination of cable “home runs” from each camera back to its recorder. Instead, a group of cameras can connect to a network switch, which connects to a network that carries the video data to viewing and recording locations. Many network encoders contain the same computer circuit boards and software that network cameras contain, and send the same types of video data packets that network cameras send. From this point on, the term “network camera” is intended to mean either an actual network camera, or an analog camera with a video signal encoded for network transmission. Many network encoders add video analytics and other network camera capabilities to analog cameras.
Server-Based Analytics
With the arrival of network cameras, NVRs were introduced. NVRs are servers that run Windows or Linux server operating systems and have a network port to accept video data from networked cameras. Some NVRs also contain analog video encoders with coaxial cable connectors, and thus support both analog cameras and network cameras. NVRs were not called “servers” for several reasons. First, manufacturers wanted customers to consider NVRs as the modern replacement for DVRs. It simplified the sales process. Second, some NVRs did not expose the end user to the server operating system software. Such NVR user interfaces were very much like the DVR user interfaces. Third, NVR manufacturers’ business models included selling the hardware and software both, for reasons of profit, quality assurance and technical support feasibility. It is simpler and easier to provide technical support for a product with operating system software that is a specific version with a specific configuration that the customer cannot alter. Thus, the NVR was a single product: video management system software provided on standard or customized server hardware.
As users became more technically sophisticated, and as IT departments began assuming management of physical security system computers, many manufacturers of video management system software unbundled their software from the server hardware, allowing customers to choose their own servers. As the pace of server technology development increased, and as server pricing decreased, many manufacturers found that providing a serverbased system on standard hardware rather than custom hardware was a more cost-effective approach. Around this time the term “video management server” came into use.
As can be seen in Figure 1, the appliance-based architecture does not scale up well to a system with a large number of cameras. It works very well, for example, for a local general aviation airport to cover the areas where private planes are parked. But it is not a feasible approach for an international airport with several thousand cameras.
Figure 2. Example of server-based analytics architecture Server-Based Architecture
Early server-based analytics systems could run simple analytics on live video streams in real time, and also on recorded video. Those searches were limited to one analytic per video stream, such as motion-detected, line-crossed, vehicle wrong-direction, and so on. Except for alarms and event information generated on real-time video streams, no analytics metadata was recorded. When running searches on recorded video, users could set new search parameters for improved searching, but each new search required re-processing the video stream. Running multiple analytics searches on a single video stream was technically possible, but was so processor-intensive that it was not practical.
Some video management system software includes simple analytics capabilities, but their application is limited to just a few video streams, with the server’s processor power being the limiting factor on how many video streams analytics can be applied to. That is why separate video analytics servers came into use.
Camera-Based Analytics
Camera-based analytics were introduced for network cameras in 1998, providing motion-based alerting and the ability to have different frame rate and image resolution settings when motion is detected in specific areas of the camera’s field of view. It became possible to transmit video images at low frame rates and/or low image resolutions to conserve recording space during times of no activity, and transmit at a high frame rate and/or high resolution during times of increased activity. Initially motion event data was transmitted to the video management server along with the video image stream, but no other image content information was extracted from the video.
Figure 3. Example of camera-based analytics architectureCamera-Based Architecture
Now (about 15 years later), network camera analytics are much more advanced, and analytics software for cameras is available from manufacturers of cameras, makers of video management systems and video analytics companies.
Cloud-Based Analytics
High levels of cloud-based computer processing power are much less costly than establishing the same high levels of computing power on facility premises, due in large part to cloud computing’s subscription-based model. To date, the primary impediment to the use of cloud-based analytics is the fact that streaming high-resolution, high-frame-rate video to a cloud services provider is not cost-feasible. However, many security video analytics do not require high-resolution or high-frame-rate video streams, and thus, residential and small business security service providers include basic video analytics in their cloud-based surveillance offerings. At least one cloud-based analytics-only offering exists, providing simple analytics based upon 320 x 240 resolution video streams. Its usage to-date has been chiefly by government and military security video analytics applications.
The arrival of distributed analytics processing capabilities has opened up new opportunities for cloud-based video analytics under the hybrid architecture model.
Hybrid Analytics
What makes the hybrid architecture possible, is that new generation video analytics supports distributed analytics processing. This is a significant breakthrough. Video analytics processing has always had two steps: image information extraction and image information analysis. Until recently, the extraction and analysis steps were always performed together with a single set of software functions.
Separating the image information extraction work from the information analysis work, and enabling the analysis work to be performed on a separate device, brings the following beneficial changes:
- Greater and more detailed image information extraction can be performed on the device processing the video streams, because its processing is confined to processing the video.
- Transmitting the extracted image information requires much less network bandwidth than video streams require, as the image information from hundreds of cameras is smaller than the video stream data from a single camera. This makes performing the information analysis work in the cloud — or anywhere — technically and financially feasible.
- The extracted image information can be fed to multiple analytics processes for parallel processing, significantly expanding the real-time situation analysis capabilities of analytics systems. A server that could formerly handle analytics for only 16 video cameras can now handle analytics for 100 to 200 cameras. As computer technologies continue to advance, the number of cameras that a single computer can process will continue to increase.
Figure 4. Example of hybrid video analytics architecture
Hybrid Architecture
- Performing video analytics searches on stored extracted image information, rather than on stored video streams, means that searches formerly taking hours can be performed in a few minutes.
- Years of extracted information from high-megapixel video images can be stored in the space formerly required by just a month or two of high-resolution video streams. This means that storing years’ worth of video-based information about customer, vehicle and other important types of activity is completely feasible — such as for retail stores, mass transportation facilities, roadway traffic patterns, insect crop activity and much more. This data can be mined to obtain valuable business information.
- When highly detailed image information is extracted, it can be saved without having to save the high-resolution video streams from which it came. Just for visual reference, mid-resolution and ultra-low-frame-rate video can be saved, or no video saved at all except for a single frame or two representing a particular event or activity. New types of analytics can be run on the stored image information, regardless of whether or not the original video images had been saved. This is a significant “future proofing” benefit.
- Big data storage and analysis tools currently exist, will continue to advance in capabilities and will already be a part of business information systems infrastructure. Thus, no new technology will need to be invented to perform data mining on the extracted video information.
Figure 5. Early analytics processing
Figure 6. Current-day analytics processing
Commercial video system products now exist in which image information extraction is performed on the camera, and the image information analysis is performed on a video management server or on a local or cloud-based video analytics server. This approach optimizes the use of the camera’s processing power, and provides faster performance of the image information extraction since it is performed on the raw video data prior to the camera’s compressing it and encoding it for network transmission. This period in time marks a distinct change in the capabilities and value of security video cameras and surveillance systems.
Figure 5 shows how previous generation analytics performed stream-based processing, whereby each analytic had to perform its own extraction of video image content information from the video stream. Figure 6 shows how new generation video analytics extracts the video image content information just one time and provides the extracted metadata to any number of analytics selected to process it.
More details about new generation analytics processing, video metadata and self-learning video analytics are provided in the sections that follow.
Metadata Advancement
Metadata is data about data. Video analytics produce video metadata. The extracted image information, and the results of the video image information analysis, are both metadata. They can be stored and retained for future analysis.
Figure 7. Example of video analytics metadata generation
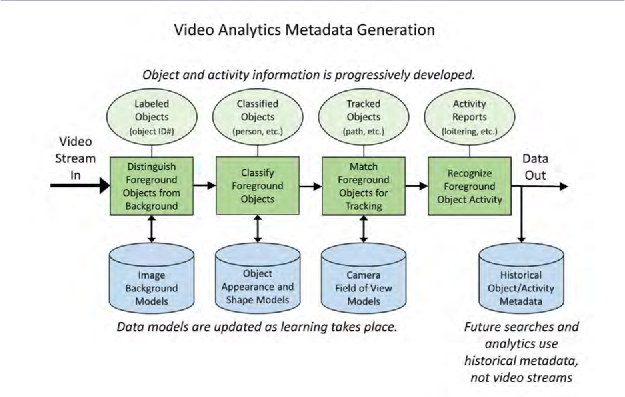
Figure 8. Example of video analytics distribute processing
In the past decade, there have been significant increases in computer chip processing power, data handling, and the number and type of video analysis algorithms. There have also been substantial improvements in video image quality, coupled with great increases in image resolution. This has allowed video analytics to advance to the point where today, metadata is highly detailed and much richer in content, making it very worthwhile to obtain and store the metadata. Searches for specific video content, such as “a bald person in a yellow shirt and brown pants”, use metadata and do not have to reprocess video streams. Thus searches can be completed almost instantaneously. This makes searching recorded video across long periods of time, and searching across multiple cameras simultaneously, quick and effective.
Figure 7 shows how comprehensive metadata generation is obtained. The better the metadata, the better the search capabilities become. Metadata analysis is the heart of video analytics, and is what generates the security and business information from the visual data that is captured by video cameras.
The role of metadata in previous generations of analytics was simply to communicate the results of the analytic process. In the new generation of analytics, metadata has two roles: to be a repository of an abundance of salient details from which new insights can be obtained, and to support the self-learning capabilities of advanced analytics systems.
Accelerating Trend in Error Rate Reduction
There are two video analytics technology trends that are effectively reducing the traditionally high error rate of the previous generations of security video analytics technologies: machine-leaning and human-assisted deep learning. Thanks to high-resolution security cameras, advanced analytics capabilities in image information extraction, and the availability of big data technologies for performing storing and processing of video metadata, a technology basis has been established for implementing automated learning in video systems.
Machine Learning
Today’s generation of video analytics are machine-learning based. Machine learning involves algorithms that can learn from and make predictions on data. Such algorithms operate by building models from an example training set of initial observations, in order to make datadriven predictions or decisions rather than follow unvarying computer program instructions. Refer again to Figure 7 to see three of the common data models that analytics systems use to continually learn and improve the quality of the analytics results.
One of the significant challenges of previous generations of analytics is that the target environment often changes, requiring the analytics to be returned for the changed environment. Analytics based upon machine learning continually update their data models to take into account environmental changes that are “normal.” For example, a typical problem with outdoor video and motion detection is that the motion masks set up during installation become outdated as trees and other greenery grow. This can cause motion-based video recording to suddenly leap to high levels and exceed the disk space allotted for it. Self-learning analytics can take this into account, and even provide alerts when the degree of change has exceeded a certain threshold.
“Machine learning is the science of getting computers to act without being explicitly programmed. In the past decade, machine learning has given us self-driving cars, practical speech recognition, effective web search and a vastly improved understanding of the human genome.”6 Business analytics software maker SAS defines machine learning this way:
Machine learning is a method of data analysis that automates analytical model building. Using algorithms that iteratively learn from data, machine learning allows computers to find hidden insights without being explicitly programmed where to look.7
Human-Assisted Deep Learning
Sometimes referred to as learning by example, human-assisted deep learning is another way to reduce the false alarm rate. Rather than decreasing analytics sensitivity to reduce false alarms, human feedback trains the analytics system, increasing the accuracy of the analytics used to determine which alarms are real and which are false to further improve a low false-positive alarm rate.
Over time, the system learns the scene and is able to prioritize important events based on user feedback. This increases sensitivity to conditions that are of concern while reducing false alarms to keep the focus on what matters. Visual search refinement can be both an aide to getting search results quicker, and a means of training the system for the kinds of searches that are needed.
Visual Search Refinement
Current analytics capabilities include human-assisted incremental visual search refinement. The following example search scenario best presents the value of metadata-based search capabilities.
The initial search increment is performed for a short period in time and selection of likely cameras, for the “person in a yellow shirt.” In a matter of seconds, 50 results can be displayed, only 10 of which are the intended subject. The search operator can visually select those 10 results, and the metadata from those results is analyzed to refine the internal system search criteria, so that the search continues even more quickly and with much more precision. This is useful, for example, at an airport to locate an individual who has left a package behind in an airport terminal. Because the system contains metadata about the cameras that cover hallways and other pedestrian paths throughout the terminal, the search can configure itself to search possible pathways, rather than having to search all cameras. Within a few minutes, the system can display a map showing a timestamped route of the individual, and the individual’s last known and possibly current location. This type of performance — and this level of capability — is only possible through the use of metadata.
Currently there are smart cameras with analytics that process raw video data as it comes from the sensor, which is faster processing because it happens before the video is encoded for compression. The search metadata is then sent to the video system for recording along with the video stream. The video from several thousand cameras can be processed in real time and the metadata recorded, without taxing the video servers. Furthermore — for self-learning systems — alerts from cameras can automatically trigger several search actions based upon past operator actions. Multiple server searches can be automatically initiated, presenting the operator with the reason for, and objective of, each automated search, and prompting the operator for search refinement to obtain the fastest and most accurate results. This is emerging technology.
Current research includes cameras reporting alert situations not just to the server but to other cameras. This is useful, for example, to continue the path identification of an individual with anomalous behavior. The behavior is detected by one camera, and the knowledge (metadata) of the individual and the behavior are passed along to other cameras, which continue recording situation-specific metadata that is also sent to the server and, as appropriate, to other cameras. Such an auto-search capability provides real-time situation tracking, and will result in security being notified of the current location and movement path of one or more individuals who can be intercepted while still on the property. In this kind of situation, eliminating 5 minutes of manually initiated search time can make a significant difference in the outcome of the situation. Such systems can be programmed to push out text descriptions of the situation along with the best identification-useful images, plus a live video feed from cameras with fields of view containing the search subject, to the responders whom the system knows are on the individual’s travel path. Consider also the fact that such a system could automatically perform a reverse-direction historical search to determine where and how the individual entered the facility, and the location of the individual’s parked vehicle if there is one. The combination of computing and networking advances, along with ongoing search algorithm and metadata R&D, continues to produce analytics capabilities that increase the span of operations a single human operator can perform.
The Video Analytics Spectrum
Many aspects of video management systems and video analytics technology are benefiting from the analytics advances. Thanks to the advanced uses of metadata in analytics, multiple analytics functions can be combined. For example, the stored metadata from multiple analytics can be processed in parallel for on-screen display, because the processing is made feasible by working with metadata and not having to reprocess the original video streams. Specific aspects about activity occurring in a portion of video can be used to specify, for example, that only vehicles or people exhibiting certain behavior be displayed. The paths of people exiting a crowd could be viewed. The paths of people who have entered a specific vehicle can be viewed in reverse. This means that it is not only the value and function of individual analytics that must be considered, but also the value of various sets of analytics combined.
Video Analytics Data as a Highly Valued Asset
As shown Figure 7, video analytics algorithms compare object and activity information against data models to perform their analysis, and update the data models based upon what is learned. This makes each analytics system’s metadata database of increasingly greater value the longer the system operates. For many organizations, security video analytics metadata is also where tremendous business intelligence value resides, especially in retail operations. Security practitioners must begin thinking about their video analytics systems not just as security tools, but as business value generators. This has major implications with regard to system cybersecurity, data privacy, data confidentiality, and policy governing the scope and use of the data.
Video Analytics and Big Data Mining
When the term “big data” came into prominence in the business world, some people in the security industry began touting security video as big data. However, security video at that time was only potentially big data. Visual information was being captured and stored, but there was no means to unlock the data it contained. That situation has changed with the new generation of video analytics technology and its metadata-based architecture. For many large organizations, video surveillance video records now constitute a valuable source of operations related information that can be mined using big-data tools, and this is an ongoing focus of video analytics research.
Video Analytics Standards and Guidance Development
There are three standards initiatives addressing industry needs with regard to security video analytics:
- Physical Security Interoperability Alliance (PSIA)
- Open Network Video Interface Forum (ONVIF)
- Video Analytics in Public Safety (VAPS)
Physical Security Interoperability Alliance
The PSIA is a global consortium of physical security providers focused on promoting the interoperability of IP-enabled devices. Its video analytics specification enables video analytics to more easily and consistently integrate with video management systems and physical security software platforms through standard interfaces.
The specification defines a standard way to share video analytics capabilities supported by an intelligent device, and output, receive, store and use various video analytic events. The open interface addresses event output including security alerts, counting events and analytics system health messages. The interface also supports the streaming of object metadata output, which includes foundational analytic output regarding all objects tracked by the analytics, including object classification, bounding box data and velocities. It is likely that this specification will be updated to account for the recent advances in video analytics technology.
Open Network Video Interface Forum
ONVIF is another leading standardization initiative for IP-based physical security products. ONVIF has a Core Specification, which covers video storage devices and video analytics engines, in addition to cameras and encoders. The release that defines standardized interfaces includes an interface for video analytics devices covering the configuration of dedicated video analytics units. ONVIF has also released a Video Analytics Service Specification, which defines the web service interface for configuration and operation of video analytics. It recognizes that video analytics applications are divided into two parts, image analysis and application specific elements. This is consistent with the evolution of new generation analytics and distributed analytics processing. However, at the time of its writing, the current generation of analytics products did not yet exist. It is likely that this specification will be updated in the near future to take into account the advanced architectures and distributed processing capabilities that have been developed.
Video Analytics in Public Safety
VAPS is a newly formed initiative, with sponsors including the Department of Homeland Security’s Science and Technology Directorate. VAPS collaborative activities are intended to inform U.S. national strategy for video analytics R&D and to lay the foundation for forming a multidisciplinary VAPS Community of Interest. It is a parallel and complementary initiative to the Video Quality in Public Safety (VQiPS) initiative, which has developed a number of highly useful guidance and educational materials that assist the first response community in clearly defining and communicating video quality needs.
In announcing the first workshop on VAPS, NIST stated, “Public safety video analytics research, development, and standards activities have lagged behind such developments in other domains. Given the explosion of video in public safety, the strategic incorporation of next-generation video analytics into public safety systems and workflows is critically important. Video analytics will play an essential role at the collection devices, in the public safety communication networks, in the data management back ends, and in real-time interactions across a variety of stakeholders and automated systems that span agencies, jurisdictions, and sectors.”8
Expect a number of valuable work products to begin appearing from the VAPS initiative in 2017.
Evaluating Video Analytics Technologies
The ultimate objective in evaluating video analytics technologies is to determine whether or not the product’s analytics capabilities will work effectively in the target environment with the video surveillance system intended for use. A good initial step is to provide the vendor with still images of the camera fields of view that the analytic must process, including some of the objects and conditions to be detected. If the vendor sees no issues of concern about the target environments, a next step can be to provide the vendor with sample video clips from various times of day that span the range of lighting and activity conditions that will be encountered. The vendor should be able to process the video clips using the selected analytics and demonstrate the results. For self-learning analytics systems, additional training footage may need to be provided.
Ask about the following:
- Which field conditions, if any, can nullify the analytic? For example, what effect will heavy rain or snow have? Are there crowding or heavy traffic conditions under which the analytic will not be able to achieve typical results?
What is the commonly experienced rate of false detections or matches, and failures to detect or match, in similar applications? - What are the various combinations of analytics that can be utilized together, and how are they used to gain insight into object and people activity and conditions that have occurred?
- What customer references are available that have similar applications, and are they willing to engage in discussions or demonstrations? What was the level of customer participation in analytic setup or tuning?
- What are the self-learning capabilities of the product? In what ways does the self-learning functionality apply to your application?
Customer references can be an important factor for analytics that require a considerable amount of setup and tuning work. For a self-learning analytic, or an analytic that works “right out of the box” for 90 percent of applications, the degree of setup effort required is not a concern.
Performing a 30- or 60-day pilot test should provide sufficient information for final decisionmaking. The test should be conducted during a calendar period of time in which the “worst case” conditions should occur. Depending upon the location, for outdoor analytics that may be a seasonal issue. For schools, the summer vacation period may not provide sufficient activity.
For actions requiring human involvement, such as locating or tracking a person or vehicle, or performing a search with visual confirmation of matches, how quickly can the actions be performed? What is the visual search refinement experience? Can searches for on-property/infacility individuals be performed quickly enough for your operational needs?
Create a set of test scenarios. If the scenarios don’t occur soon enough through normal activity, stage the activity by getting together a small group to create the conditions that the analytics product should detect and respond to.
It is important to develop acceptance criteria — what will success look like with regard to your use of the analytics system?
Know that some analytics will work better than others, and not all new generation video analytics will work perfectly. A key question is: Will they work well enough to be useful in the ways that you intend?
Most vendors with analytics products will advance their technology on a regular basis. Find out about their future plans, and ask to see their technology roadmap (this may require a nondisclosure agreement). Most companies do their best to leapfrog ahead of the competition, which means that at any time, a competitor may introduce an analytic product or capability that surpasses the one that you have in place. Remember that your own product may advance and reverse the situation. In general, the entire field of analytics is advancing and so should any product that you select for deployment.
If cloud analytics services are involved, be sure that the service level agreements (SLA) that both you and your security integrator (reseller) receive are consistent with each other and fully satisfactory. It may be prudent to use a hybrid architecture, in which critical real-time analytics are run on a local server, and less critical analytics are run in the cloud.
The Future
The future for security video analytics is being defined around the globe by advanced video research occurring today. One example is light field technology, which models a point of light as having not only a three-dimensional location in space, plus color and brightness, but also having a direction and speed of travel. Wikipedia states, “One type of light field camera uses an array of micro-lenses placed in front of an otherwise conventional image sensor to sense intensity, color, and directional information. Multi-camera arrays are another type of light field camera. Holograms are a type of film-based light field image.”9 Note the use of the word “models” in the description of the technology, which is metadata-based. When a light field camera performs image extraction, it captures 2D, 3D and time information, much more information than security video analytics technology captures now. (For very technical information see the Wikipedia articles on Light Field10 and Light-Field Camera. 11)
This multi-dimensional information allows light field camera images to be focused after the images are taken. Lytro (www.lytro.com), a manufacturer of light field cameras, provide an online gallery of pictures that you can focus after the fact online. Amazon sells the Lytro Illum camera kit currently for around $470.
Light field capabilities are of high interest to filmmakers because the metadata from the visual images maps to metadata used in animated 3-D filmmaking and computer graphics animation. This allows visual film images to be seamlessly combined with computer graphics animation more quickly and easily than can be done with traditional animation technologies. It also allows the use of light field video for both 2D and 3D gaming and virtual reality applications.
However, it is not just in metadata capabilities that light field cinematography excels.
For example, the Lytro Cinema12 camera is a professional camera that captures 750megapixel images at 300 frames per second. It allows after-shoot refocus plus a small amount of 3-D rotation capability.
These capabilities far exceed any currently imagined requirements of security video. Think of how easily a video surveillance system could track people and objects through, for example, an airport, if it had 3-D coordinates plus direction of movement information for each person and object, and if the entire facility existed as a 3-D model that could be visually presented and rotated for an optimum 3-D view on playback or in real-time tracking. That is the future direction of video technology — and all of its underlying technology — under development now.
Conclusion
The capabilities of new-generation video analytics technology already go well beyond the earlier promises of video analytics performance. With the continuing advancement of computer-related technologies, video analytics remains an area of continual and rapid advancement. Going forward, video analytics will become a greater and more integral part of our risk mitigation technologies.
Many of these advances will come from initiatives outside the security industry. However, as futuristic as the visions of many technologies seem now, our selection and deployment of these technologies will remain grounded in the principles that are familiar to us. Our decisions will be based upon the role that the technologies can play in achieving our objectives for safe and secure facilities, services, events and activities.
Ray Bernard (RayBernard@go-rbcs.com) is president and principal consultant of Ray Bernard Consulting Services (www.go-rbcs.com).
References
1. Nik Gagnani, “Introduction to video analytics,” EE Times, 22 Aug 2008, web, accessed 05 Jul 2016.
2. “artificial intelligence.” Dictionary.com Unabridged. Random House, Inc. 17 Jul. 2016.
Dictionary.com http://www.dictionary.com/browse/artificial-intelligence
3.“artificial intelligence.” The American Heritage® Science Dictionary. Houghton Mifflin Company. 17 Jul. 2016
Dictionary.com http://www.dictionary.com/browse/artificial-intelligence
4. Bernhard Rinner, “Video Surveillance: Past, Present, and Now the Future,” IEEE Signal Magazine, IEEE, May 2013: 198. Print.
5. “architecture.” Oxford Dictionaries. Oxford University Press. 17 Jul. 2016.
6. Andrew Ng, “Machine Learning”, from the description of the Stanford University online course, 18 July 2016,
coursera.org https://www.coursera.org/learn/machine-learning
7. “Machine Learning: What it is & why it matters.”, SAS. 18 July 2016,
sas.com http://www.sas.com/en_id/insights/analytics/machine-learning.html
8. “The First Workshop on Video Analytics in Public Safety (VAPS)”, NIST.
nist.gov http://www.nist.gov/itl/iad/2016-workshop-on-video-analytics-in-public-safety.cfm
9. Wikipedia contributors. “Light-field camera.” Wikipedia, The Free Encyclopedia. Wikipedia, The Free Encyclopedia, 14 Mar. 2016. Web. 22 Jul. 2016.
10. Wikipedia contributors. “Light field.” Wikipedia, The Free Encyclopedia. Wikipedia, The Free Encyclopedia, 12 Apr. 2016. Web. 22 Jul. 2016
Wikipedia.org https://en.wikipedia.org/wiki/Light_field
11. Wikipedia contributors. “Light-field camera.” https://en.wikipedia.org/wiki/Light-field_camera.
12. “Lytro Cinema”, Lytro. 22 Jul 2016.