Quality In, Accuracy Out: Applying Video Analytics to High-Quality Images for Effectiveness
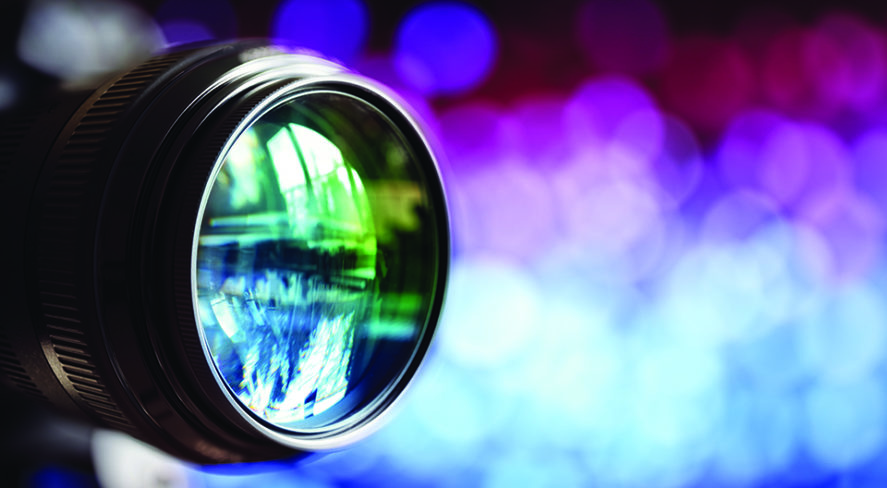
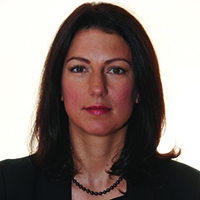
For the past 15 years, the accuracy and range of video analytics have tremendously improved, from basic people counting to facial recognition. Indeed, what was yesterday’s imprecise line crossing has given way to artificial intelligence (AI) and deep learning-based people and object recognition.
Several factors directly affect the precision and efficiency of these applications, including processing power, algorithms and – one that is frequently undervalued – image quality. It is important to remember that, as with any other type of data analysis, video analytics depend on accurate input to provide reliable results and reduce false positives and false negatives as much as possible. The most powerful processor and the most sophisticated algorithms will still produce erroneous findings if the image quality is poor.
Video Analytics in Brief
Depending on the desired usage and the security surveillance system configuration, analytics can be done at the video management system (VMS) level, the network video recorder (NVR) level or the camera level. While some post-processing analysis is performed, most of today’s analytics are done in real time, whether centrally or at the edge, delivering information to users as events occur. Furthermore, with the power of processors rising and their costs decreasing, more complex analytics, including AI and deep learning, can now be achieved at the edge, providing more system flexibility and efficiency. As such, several equipment manufacturers have cameras with various analytics suites, either for multiple purposes or for specific applications such as license plate recognition and facial recognition. With this increase in complexity, image quality becomes of paramount importance to ensure that the correct information is captured, analyzed and learned by the system.
Video analytics are based on rules set by the user. When these rules are met, a notification is sent. A simple rule could involve, say, a person crossing a specific area or a piece of luggage left unattended. More complex rules could, for example, identify all black cars in a certain zone or all people who are carrying a red backpack. To carry out simple rules, the system will apply specific algorithms. These algorithms analyze the image captured by the camera – that is, the pixels composing the image – to determine if the conditions specified by the rule are met or not. Typically, at this level of complexity, the algorithms search for certain defined changes in pixels within a specific zone of the image and within a certain timeframe.
While more complex rules also use algorithms, they perform a more precise analysis of the image, which may involve eliminating irrelevant information such as foliage or animals and very often includes some machine learning. For example, for the system to recognize and understand what a black car is or what a cat is, it must be taught. In addition to deep learning techniques, there are other machine learning methods that use statistical and mathematical models. And while the choice of machine learning type usually depends on several factors, including the algorithms used and the application, the starting point is still image analysis. Even at this complexity level, a small change in some pixels may result in a false response. For example, when teaching a system about facial recognition, a discrepancy between some pixels and reality can make a trait look completely different.
Understanding Image Quality
In the context of analytics, image quality can be defined as the accuracy of an image captured by the camera. It is important to mention that, when analytics are performed, they are not affected by human perception, unlike when a display is viewed by a person under various lighting conditions. Furthermore, the analytics are performed on uncompressed images to ensure the accuracy of the input. When done at the edge, they are completed before compressing the image and transmitting it. When done in an NVR or a VMS, the image is first decompressed and then the analysis is applied.
While image quality may seem to be subjective, it is in fact possible to assign it measurable attributes. Indeed, image quality is directly correlated to the modulation transfer function (MTF) of the optical system and the f number and relative illumination (RI) of the lens. Whereas low-light performance increases as the f number decreases, high MTF and controlled RI are defined by optical designers based on the type of lens and its applications. Distortion is another attribute of image quality, and although it should be minimized in most cases, controlled distortion is an important characteristic in wide-angle cameras that maximize the magnification factor or pixel density where needed.
Principal factors affecting image quality can be divided into three categories: the optical system components of the camera and their related characteristics, the environment and human interaction.
In regard to the optical system, near perfect image quality can be achieved by pairing up a high-quality lens with an appropriate sensor. If the lens is of low quality, the image will be as well, regardless of the sensor’s specifications and performance. In addition, the entire image processing pipeline has a direct effect on image quality: A camera that has a high-quality lens, sensor, image signal processor and encoder will still display poor images if the image processing is inadequate.
Probably the best-known image quality factor related to the sensor is the image resolution/pixel count. With multi-megapixel cameras now common, state of the art image quality should be achieved. However, the effects caused by digital zooming must be accounted for. As the camera zooms digitally within an area to analyze it, pixels are interpolated, the resolution decreases and the image quality may suffer, especially for cameras with lower resolutions, such as 1.3 megapixels.
Furthermore, the camera angle of view directly influences the image quality and is correlated to the resolution, as well as the type of lens used. For angles of view of less than 80 degrees, the pixel density is generally rectilinear across the entire image, and the quality is mostly constant, with a little MTF drop toward the edges. With conventional lenses, as the angle of view increases, the pixel density tries to remain constant up to a decrease at the edges. This is a well-known challenge for wide-angle cameras using a traditional fish-eye lens, which displays significant distortion at the edge of an image, where the pixel density is lower than at the center. In these cases, the image quality in the center is adequate for analytics processing, but closer to the edge, it is not. Alternatively, wide and ultra-wide cameras equipped with panomorph lenses generate less distortion at the image’s edge, because of a higher pixel density, which provides sufficient quality for analytics processing throughout the image. Figure 1 demonstrates the difference between a traditional fish-eye lens and a panomorph. On the edge of the fish-eye lens image, one can observe a simple black rectangle, whereas in the same zone with the panomorph lens, one can clearly see a computer screen.
Other important factors that affect image quality are related to the environment, with lighting conditions having the most direct effect. While some surveillance occurs under constant lighting conditions, a large proportion takes place in light that varies. Bright light and low light can affect how a camera captures a scene, producing over or under-exposed images, which results in inferior image quality for analytics processing. To mitigate the impact of bright and low light on image quality, cameras with wide dynamic range are highly recommended. Similarly, for very low-light conditions, such as at night, cameras with infrared sensors are good options.
Additional environmental factors that influence image quality are the ambient temperature and the humidity level. Extreme heat and cold, abrupt temperature changes, and high humidity can hinder the functioning of the camera sensor and processor, as well as the camera focus adjustment, all of which affect image quality. Also, mist may form on the camera bubble, degrading visibility. Where weather factors are a concern, ruggedized and extended temperature cameras with autofocus mechanisms, heaters and ventilated housings should be considered.
Lastly, a highly variable type of factor in image quality is human interaction, primarily through the installation and configuration of the cameras. While camera configuration is becoming more automated, it still has a human element that can influence image quality. Proper physical mounting and alignment and accurate setup of all parameters, including focus, are important steps to ensure sharp images. Special attention should be paid to the impact of varying lighting conditions to ensure that the parameters address all of the conditions mentioned above. Consequently, it is of vital importance to make sure that the technicians installing the cameras are properly trained.
Summary
Tremendous advances have been made in the field of video analytics, especially with the use of AI and deep learning, which allow for sophisticated rules and analyses, whether handled at the edge, on an NVR, or in a VMS. As the complexity of analytics increases, so does the need for images to be of excellent quality. To ensure high image quality, several aspects must be considered: the design of the entire optical system and imaging pipeline, the environment and the human factor. Thus, as for any process, the quality of the input directly affects the accuracy of the output.
While the lens-to-display pipeline has shifted from analog to digital and has been improved by new technologies, it has remained centered on the expectation that humans are looking at the images. When the human observer is removed, as in the analytics process, the entire imaging pipeline must be revisited to accommodate the new technical demands and enable AI to open up new areas of innovation.
Sophie Gigliotti is manager, sales and business development, video security for Immervision.