What Science Really Says About Facial Recognition Accuracy and Bias Concerns
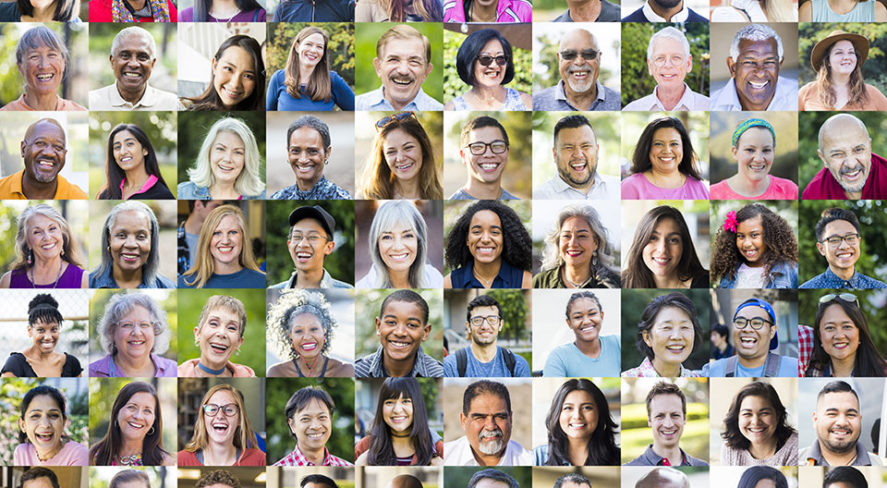
Updated March 11, 2024
Since 2020, calls to heavily restrict or ban the use of facial recognition technology have become commonplace in technology, data privacy and artificial intelligence policymaking discussions. In many cases, the sole justification offered is the claim that this face matching technology is generally inaccurate, and particularly so with respect to women and minorities.
Surely, a technology used in identification processes with significant outcomes should perform well and consistently across demographic groups. But does science really support claims of inherent “bias”? What is the evidence being cited for this claim, and does it add up?
First, we must understand that whether it’s face, fingerprint, iris or another identification modality, no biometric technology is accurate in matching 100% of the time, and there will always be an error rate, however small. Performance for each modality can be studied scientifically. And this performance is affected by different factors, the most significant of which for facial recognition involve capture conditions like camera focus, field of depth, blur, off-angle positioning, etc.
Massive improvements in the performance of facial recognition technology in recent years are well documented, and this performance is what is driving widespread adoption across government, commercial and consumer applications. But what does scientific research say about the performance of facial recognition technology today? The most reliable data available shows that a large number of leading technologies used in commercial and government applications today are well over 99% accurate overall and more than 97.5% accurate across more than 70 different demographic variables, as we explain in more detail below.
It’s important to put this into context and understand this accuracy reaches and exceeds the performance of leading automated fingerprint technologies generally viewed as the gold standard for identification, which, according to U.S. government technical evaluations, achieve accuracy rates ranging from 98.1% to 99.9%, depending on the method of submission. This accuracy also reaches the accuracy levels of leading iris technologies, which ranges from 99% to 99.8%. according to U.S. government evaluation.
While there is evidence that older generations of facial recognition technology have struggled to perform consistently across various demographic factors, the oft-repeated claim that facial recognition is inherently less accurate in matching photos of Black and female subjects is simply false. In fact, the evidence most cited by proponents of banning or heavily restricting facial recognition technology is either irrelevant, obsolete, nonscientific or misrepresented. Let’s take a look.
The Problem With MIT’s “Gender Shades” Study
By far, the source most cited by media and policymakers as evidence of bias in facial recognition is Gender Shades, a paper published in 2018 by a grad student researcher at MIT Media Lab, a self-described “antidisciplinary research laboratory, growing out of MIT’s Architecture Machine Group in the School of Architecture.” Heralded in many media reports as “groundbreaking” research on the bias in facial recognition, the paper is frequently cited as showing that facial recognition software “misidentifies” dark-skinned women nearly 35% of the time. But there’s a problem: Gender Shades evaluated demographic-labeling algorithms not facial recognition. Specifically, the study evaluated technology that analyzes demographic characteristics (is this a man or a woman?), which is distinctly different from facial recognition algorithms that match photos for identification (is this the same person?).
A second problem is that the algorithms evaluated were those publicly available in 2017 (now quite old given the pace of innovation in computer vision). Basically, what we learn from the Gender Shades study is that software from IBM and several others was not very good at face/gender classification when tested in 2017. IBM immediately challenged even that limited result, saying that its replication of the study suggested an error rate of 3.5%, not 35%. Most importantly, the report says nothing about the accuracy of facial recognition technology, yet it is often the sole data point cited in claims that facial recognition is rampantly inaccurate when it comes to persons of color. Misconstruing demographic labeling technology as facial recognition has continued with false claims that the technology is “even less reliable identifying transgender individuals and entirely inaccurate when used on nonbinary people,” based on tests of classification software that again do not involve identification.
ACLU’s Intentionally Skewed 2018 Test
Also frequently cited by critics of facial recognition technology is a 2018 blog post by the American Civil Liberties Union (ACLU) regarding a test it claimed to have performed using Amazon Rekognition, a commercially available cloud-based tool that includes facial recognition. According to the ACLU, it created a database of 25,000 publicly available arrest photos and ran a search of these using official photos of the 535 members of Congress, returning “false matches” for 28 of them. The ACLU claimed that since 11 of these matches, or 40%, were people of color, and only 20% of Congress overall are people of color, this is evidence of racial bias in facial recognition systems; however, the search results were returned using a “confidence level” of only 80%, which returns more possible match candidates with lower similarity scores for the human user to review. Even if the ACLU test were to have replicated typical law enforcement investigative uses by having the software return to the operator a set number of top-scoring potential matches, the ACLU 1) does not report the ranking or score of the nonmatching photos and 2) does not indicate there were any authentic images of the members of Congress included in the database for the software to return. So of course, any possible match candidates returned at that low threshold from a database consisting only of arrest photos were nonmatching individuals with prior arrests.
This nonscientific and misleading test was clearly performed and interpreted to provide a desired result. Amazon later conducted a similar test of its software using a 99% confidence threshold (which it recommended for actual law enforcement investigative usage) against a vastly larger and more diverse data set of 850,000 images – reporting the software returned zero “false matches” for members of Congress; however, the ACLU continued to publish additional results using state legislator, celebrity and athlete photos, using its same flawed test methods errantly claiming the results indicate the accuracy of facial recognition technology.
A 2012 FBI Study Analyzing Now-Obsolete Algorithms
A more-than-decade-old study involving researchers from both the Federal Bureau of Investigation (FBI) and the biometrics industry has also been frequently cited to support bias claims. In this evaluation, several algorithms available at the time were 5-10% less likely to retrieve a matching photo of a Black individual from a database compared to other demographic groups; however, the now-obsolete algorithms in the study predate the deep learning technologies enabling the thousandfold increase in facial recognition accuracy since that time. For example, 10 years ago, accuracy was measured in errors per thousand candidates versus per million today – Stone Age versus Space Age tools. Despite the irrelevance of the study today, it continues to be cited as a primary source for claims of racial bias facial recognition technology, a fact bemoaned by its author, who points out that it was “incomplete on the subject and not sufficient” to support such claims even at that time.
2019 NIST Demographic Effects Report
For more than 20 years, the National Institute of Standards and Technology (NIST) Facial Recognition Technology Evaluation (FRTE), which was previously known as the Facial Recognition Vendor Test (FRVT), has been the world’s most respected evaluator of facial recognition algorithms – examining technologies voluntarily provided by developers for independent testing and publication of results. But even NIST’s most significant work has been continually misrepresented in policy debates.
In 2019, NIST published its first comprehensive report on the performance of facial recognition algorithms specifically across race, gender and other demographic characteristics (“demographic differentials”). Importantly, the report found that the leading top-tier facial recognition technologies had “undetectable” differences in accuracy across racial groups, after rigorous tests against millions of images. Many of the same suppliers are also relied upon for the most well-known U.S. government applications, including the FBI Criminal Justice Information Services Division and U.S. Customs and Border Protection’s (CBP’s) Traveler Verification Service.
Lower-performing algorithms among nearly 200 tested by NIST at that point in time in 2019 – many of which were academic algorithms or in various stages of research and development rather than widely deployed commercial products – did show measurable differences of several percentage points in accuracy across demographics. Critics completely ignored results that showed demographic differences are a solved problem for the highest-performing algorithms, seizing instead upon the lowest performers, claiming the report showed “African American people were up to 100 times more likely to be misidentified than white men.”
It has further become clear that outliers from fraudulent Somalian data is responsible for much of the reported difference. This part of the evaluation relied on foreign visa application data to establish ground truth, ignoring the risk of visa fraud in those applications. Higher levels of fraud in the data set means images of the same individual are erroneously labeled as belonging to different people. As a result, the report found a much higher match error, in particular for visa applicants from the failed state of Somalia, where visa fraud is particularly problematic. In reality the algorithms were properly identifying fraud where the same person applies for visas under multiple names. This information helps explain why, outside of data from Somalia, nearly all other country-to-country comparisons across algorithms yielded much higher accuracy rates in the report (see SIA’s analysis), and it is the reason why this data from Somalia has now been excluded from NIST’s ongoing evaluation (see below).
Ongoing NIST Facial Recognition Vendor Test Program
While the 2019 NIST demographic report provided a moment-in-time snapshot, NIST began publishing a demographic differentials update along with its FRTE 1:1 monthly report nearly four years later in July 2022, as part of its Ongoing program that now evaluates more than 550 algorithms on an regular basis.
This update provided 12 “false match” and 8 “false nonmatch” measurements of performance on a vendor-by-vendor basis to encourage further innovation and improvements with respect demographic differentials; however, some developer concerns about potential data mislabeling/ID fraud remain, as the test data set for this part of the evaluation continues to derive from visa applications submitted by foreign embassies. In addition, since information on the race associated with each image is not available, the evaluation of performance across racial demographics continues to be inferred based on country of origin of the image. The data set is divided into two sexes, five age groups and seven country regions as a proxy for racial groups and other demographics (for a total of 70 demographic subcategories), and most of the performance measures compare the most accurate subcategory against the least accurate subcategory (the largest possible differential).
Due to the high precision measurement of matching ability, held at the constant threshold of a one in a million false nonmatch rate on the overall population, a single error among millions of comparisons can have measurable impact on percentages. The NIST demographic differentials ongoing study further merits careful consideration because its focus on relative differentials across a dataset minced into 70 subcategories can obscure stunning accuracy improvements in absolute terms, both on an overall basis and within each subcategory.
As of Jan. 23, 2024, the highest subcategory false match rate listed on the leaderboard for the best 50 algorithms was 2.442% or better, meaning that even for the worst performing subcategory and the worst algorithm among world leading providers, accuracy remains very high, with a true reject rate greater than 97.5% at a true accept rate of 99.8%.
And at the same time, other FRTE evaluations that are part of the 1:1 report use a different set of images that do have individual race information, potentially providing a more relevant indicator of performance across key demographic factors of most interest to researchers and developers. In fact, accuracy across these demographics is very closely balanced, and if anything, the white male sub-demographic shows the lowest accuracy, not the highest.
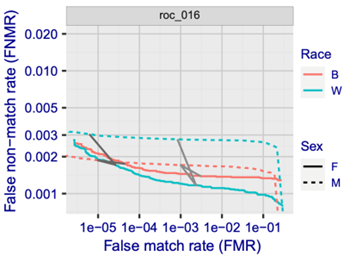
According to evaluation data from January 22, 2024, each of the top 100 algorithms are over 99.5% accurate across Black male, white male, Black female and white female demographics. For the top 60 algorithms, accuracy of the highest performing demographic versus the lowest among these varies only between 99.7% and 99.85%. Unexpectedly, white male is the lowest performing of the four demographic groups for the top 60 algorithms. (See data beginning with figure 180 on page 258. Accuracy is stated here as the true accept rate (TAR) at a set false accept rate (FAR) of roughly 10-6 or 0.0001%. Note: TAR is the inverse of false nonmatch rate, and FAR is synonymous with false match rate.)
Furthermore, this evaluation under the FRTE Ongoing program, which finds no evidence of demographic bias, uses mugshot data from US law enforcement records that has firmly established ground truth (accurately labeled data), versus foreign government-supplied visa application data, which as described in the case of Somalia can be unreliable.
Next Steps to Addressing Accuracy Concerns
While no method of scientifically testing the accuracy of facial recognition algorithms is without limitations, so far the science shows that to the extent accuracy might vary across demographic groups (i.e., “bias”), overall accuracy is at an astonishingly high level and continues to improve at a rapid pace with innovation. Even the worst performances for sub-demographic groups (relative to others) still show highly accurate results overall. And the highest-performing algorithms do not exhibit wide discrepancies in accuracy between major demographic groups.
At the same time, it is also clear that much more thorough, frequent scientific research, testing and evaluation of facial recognition technologies is necessary to both validate accuracy gains and provide tools to developers to ensure performance is consistent. For over 20 years, NIST has remained the most reliable independent, third-party scientific evaluator, providing an “apples to apples” comparison of the performance of facial recognition technologies. Despite the claims of some to the contrary, the range of ongoing evaluations conducted under the NIST program include those with direct relevance to many of the most important use cases for facial recognition technology. This includes law enforcement applications, where FRTE involves relevant types of images of varying quality (including mugshots, border crossing, webcam, and other images), relevant evaluations including “investigative performance” and queries against data sets similar or larger in size than what would be available to law enforcement agencies (up to 12 million images). Under the Investigative Performance evaluation, for example, the top 30 algorithms can successfully match a photo in a database of this size 98-99.4% of the time.
The market for facial recognition technology is global and extremely competitive, with suppliers continually working to provide technology that is as effective and accurate as possible across all types of uses, deployment settings and demographic characteristics. A product cannot be competitive if developed using nondiverse data and accuracy performance is not consistent. The U.S. government relies on NIST programs to assess technologies it uses every day in border security, international traveler verification and other applications. Algorithms used in border applications are a good example. For an application to be effective, the technology must be accurate for travelers from anywhere in the world and any racial background or demographic. CBP currently uses the technology for identity verification at 238 airports around the world, including at exit in 38 U.S. airports. To date, more than 300 million travelers have participated in the biometric facial comparison process at U.S. air, land and sea ports of entry, and CBP has intercepted more 1,800 individuals attempting to enter the country under false identities
Policymaking should focus on ensuring that facial recognition technology continues its rapid improvement, that only the most accurate technology is used in key applications and that it is used in bounded, appropriate ways that benefit society. SIA and stakeholders across multiple industries have urged Congress and the White House to provide additional resources to NIST testing and evaluation programs, allow expanded testing activities NIST has identified to more thoroughly and regularly evaluate performance of the technology across demographic variables, test the full range of available algorithms, coordinate with federal agencies that deploy facial recognition in the field and assess identification processes incorporating both facial recognition technology and trained human review.
Steps can be taken to ensure the technology is used accurately, ethically and responsibly without limiting beneficial and widely supported applications. SIA has developed policy principles that guide the commercial sector, government agencies and law enforcement on how to use facial recognition in a responsible and ethical manner, released comprehensive public opinion research on facial recognition use across specific applications and published information about beneficial uses of the technology.