Exploring AI Large Language Models – Boon or Bane for Security and Surveillance?
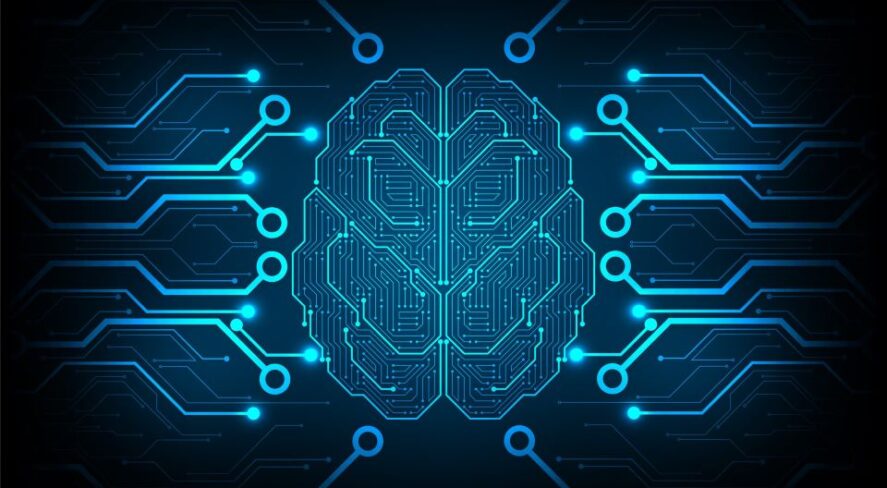
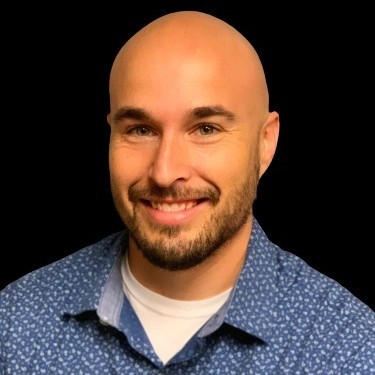
Large language models (LLMs) like ChatGPT have taken the world by storm, with ChatGPT becoming the fastest-growing app of all time. Most of us can’t make it more than a few minutes without hearing about the wonders of artificial intelligence. These conversations can be intimidating for some and uninteresting to others, so in this article I will try to break down in very simple terms what exactly LLMs are, the basics of how they work and how they might be used in our industry over the next few years.
What Are AI Large Language Models?
Let’s start by demystifying what LLMs actually are. Picture a colossal digital brain trained on a vast array of text data from books, articles, websites – you name it. This brain isn’t just repeating what it’s learned; it’s making connections, understanding contexts and generating responses that can be startlingly humanlike. That, in a nutshell, is an AI LLM.
These models are called “large” because they’re built on enormous data sets and require significant computational power. They’re the latest in a series of steps toward creating AI that can understand and interact with human language in a meaningful way.
In addition to using a model to make connections to large amounts of data, LLMs require a lot of human training. For example, if you go onto ChatGPT and ask it a question, you will notice that you can give the response a “thumbs up” or “thumbs down” indicating whether the response was good or not. This teaches the model to craft better responses, understand the context of human questions better and make different connections with the data. The models are always learning and always adapting because there are millions of people training the model to be better.
What is the difference between AI and LLM? Short and sweet, LLM is just a subset or category of AI. So, all LLMs are artificial intelligence. It’s not uncommon to hear people refer to LLMs as just AI. Perhaps a good way to think about it, is the difference between the words “automobile” and “Honda Accord.,” with automobile representing AI and Honda Accord representing LLMs. All Honda Accords are automobiles, but not all automobiles are Honda Accords. Other popular categories of AI are machine learning (ML), computer vision, and robot processing automation (RPA) to name a few. But these will be topics for a different day.
Potential Uses in Security and Surveillance
LLMs in security and surveillance are brimming with potential. Many systems in our industry are already using AI and LLMs to make their systems better. Here’s a glimpse into what they can do:
- Threat Detection and Analysis: AI system that can sift through mountains of video, access control and communication data to detect potential security threats. LLMs can analyze patterns, understand nuances and flag anything suspicious far more quickly than any human could. These systems can constantly train themselves to be better and can tie into threat detection systems to quickly recognize and adjust to emerging threats. For example, gun threat detection systems can automatically initiate lock downs and notify authorities in the event of an incident.
- Forensic Analysis: LLMs can assist in combing through evidence in criminal investigations, interpreting complex documents and generating potential leads based on analysis. Law enforcement can search through large data lakes of surveillance information using plain language search. For example, law enforcement agencies are currently using AI-driven tools for digital forensics to process vast amounts of digital evidence, like emails and text messages, to uncover patterns and clues in criminal investigations. This capability enhances the speed and accuracy of criminal investigations, enabling law enforcement to sift through digital evidence quickly and identify relevant information, which might take humans much longer to process. Often, LLMs can detect patterns that a human may have missed.
- Surveillance Monitoring: With their ability to process and understand vast amounts of textual data, LLMs can monitor communications or online activities for security purposes, potentially identifying harmful intentions or actions. AI-based surveillance systems are being used in smart cities for public safety monitoring. For instance, cities like Singapore have implemented AI systems to analyze footage from CCTV cameras across the city. These systems can recognize patterns of behavior and alert authorities to potential security risks, like unattended bags in public spaces or unusual gatherings that may indicate a security threat.
Applications in Security Operations
The impact of LLMs on our industry extend far beyond security technology – it can also revolutionize various operational aspects of security and surveillance to make people more efficient.
- Bid Response and Automation: LLMs can help enhance efficiency in responding to security-related bids and automating routine tasks. They can autofill necessary data, draft initial responses and even analyze previous successful bids to optimize new ones. A security firm could use an LLM-based system to handle numerous bids for projects. The AI can process request details, match them with the company’s capabilities, and even draft customized responses based on previous successful proposals. Such automation not only saves time, but also increases the chances of winning bids by creating more tailored and competitive proposals.
- Sales Follow-Ups: LLMs can automate follow-up communications. They can send context-aware responses to potential clients, answer FAQs and keep the sales pipeline moving efficiently. A company selling security solutions might use an LLM to manage follow-ups with leads generated at a trade show. The AI can personalize emails based on the conversation notes entered by the sales team, ensuring each lead receives a relevant and timely follow-up. This level of personalization and efficiency in follow-ups can significantly increase conversion rates and customer satisfaction.
- Mundane Task Automation: LLMs can take over repetitive tasks such as data entry, report generation and routine customer queries, freeing human teams to tackle more complex challenges. In a security operations center, an LLM might be used to generate regular incident reports, compile statistics and respond to standard client inquiries about their security systems. Automating these tasks reduces the risk of human error, enhances overall productivity and allows the human workforce to focus on strategic tasks that require human insight and decision making. Chatbots are also being employed for many customer service and tech support roles, allowing for fast communication with customers and enhanced customer service.
The Bright Side: Benefits of LLMs in Security
There is a big debate around AI and LLMs and the impact they will have on industries and society. Will they put people out of work, shift the workforce, displace some of our creative workforce, or will they signal the end of privacy as we know it? Those are all conversations worth having, but it isn’t all doom and gloom- the advantages of employing LLMs in security and surveillance are significant:
- Efficiency: LLMs can process information at a speed and scale unattainable by human teams. This efficiency can help security forces react to threats much more quickly and they can automate mundane tasks that can make our workforce more efficient.
- Accuracy: With continuous learning capabilities, LLMs can improve over time, reducing threat detection and analysis errors. This ability to learn and adjust creates systems that are getting better every second, helping to reduce false positives in our detection systems.
- Cost-Effective: Automating tasks with LLMs can reduce operational costs in the long run by making our current staff more efficient and accurate, although it should be noted that it can be quite costly to purchase or deploy custom AI systems in a production environment. So a return on investment analysis will need to be performed.
The Flip Side: Ethical and Privacy Concerns
It’s not safe to assume that it is all sunshine and rainbows either. We are not even aware of all the potential dangers that AI can introduce. The use of LLMs in security and surveillance raises important ethical and privacy concerns:
- Privacy Invasion: The capability of LLMs to analyze personal communications can lead to invasive surveillance, infringing on individual privacy rights. LLMs can ingest social media information, texts, emails, surveillance footage, access control information and any other digital record you can think of. This data can be used to aggregate a lot of personal and private information on an individual. All your habits, routines and tendencies are known to this digital brain, and in the wrong hands that could be dangerous or disruptive to our way of life.
- Bias and Discrimination: If the training data for these models is biased, the AI’s decisions and analyses could be unfairly skewed, leading to discrimination. We have to make sure that we are using unbiased data sets, identifying bias in AI and training that bias out. It is a very long and difficult process to properly train AI systems.
- Accountability: With AI making decisions, determining accountability in case of errors or misuse becomes challenging. If an AI security system makes a wrong decision, who could be held liable or accountable for that decision?
The Balancing Act
The key lies in finding a balance. Ensuring that LLMs are used responsibly in the security and surveillance sector involves:
- Clear Regulations: Our industry needs clear regulations and guidance on how to embrace AI safely, securely and ethically. The federal government is working on an AI framework that will end up applying to all AI generates in the United States.
- Transparency and Accountability: Maintaining transparency in AI operations and establishing clear lines of accountability. This includes sharing data sets, software code and AI training models to the public.
- Ethical Considerations: Prioritizing ethical considerations and privacy protections in developing and deploying these technologies. Every organization that is developing AI needs to publish and follow ethical guidelines for AI.
Conclusion: A New Frontier With Caution
As we stand on the brink of this new AI-powered era, embracing the benefits of technologies like LLMs while being acutely aware of their potential pitfalls is crucial. The future of AI in security and surveillance promises efficiency and innovation, but it must be navigated with caution, ethics and a strong commitment to the greater good.