Considerations in Artificial Intelligence Training and Deployment
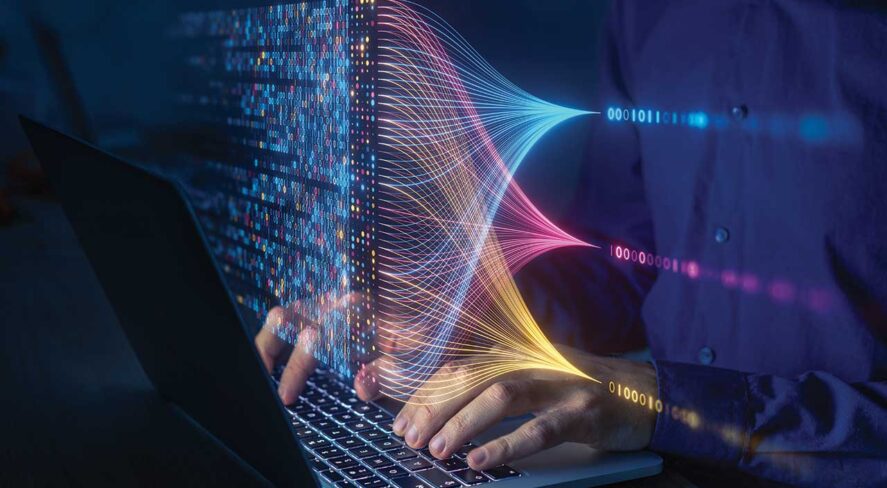
Starting with the right implementation plan is vital to success
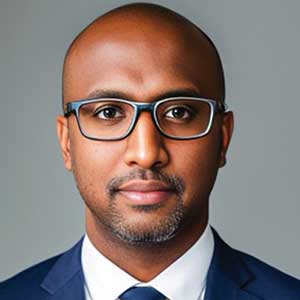
More businesses are starting to deploy artificial intelligence (AI), which means they need to understand new rules for using data properly and safely. When adding AI to their systems, especially in physical security, companies face not just technology challenges but also compliance demands that differ from state to state, industry to industry, and country to country.
An improper approach to AI deployment could put an organization’s entire security program at risk. For large enterprise customers, this process can be a part of digital transformation. For end users, it is important to think about how to get ready for the big changes that come with AI.
There are many regulations related to AI around the world that companies need to follow, and they can get in trouble if they fail to do so. When companies want to use AI, they need to be careful about these rules, especially when they are handling personal information.
The first step in a deployment is understanding how AI works with current tools. Most likely, it will not provide a significant return on investment initially but, with a well designed implementation roadmap, it may enhance efficiency or improve how security operations are managed in the future.
AI requires a massive amount of data to build its learning models, which raises multiple issues.
- Ethical and privacy concerns: Gathering large volumes of data often involves collecting personal information, posing significant privacy concerns.
- Financial costs: Acquiring high-quality, relevant data can be expensive. Costs are associated with purchasing datasets, investing in data collection infrastructure, and partnering with data providers.
- Data bias and quality issues: Large datasets are not immune to bias, which can lead to skewed AI outputs. Ensuring diversity and representativeness in datasets requires additional efforts in data curation and preprocessing.
- Legal and regulatory constraints: Data acquisition is heavily regulated, with laws varying by jurisdiction.
Addressing these challenges requires a balanced approach that considers the ethical implications of data collection, invests in data quality, and adheres to legal standards. Companies need to be transparent about their data practices and data protection. For example, processing access control event data through a large language model (LLM) would involve collecting the behavior of employees when they enter and exit the facility. If this includes information about the CEO of the company, it could create risk if the data is acquired by bad actors. Banks, hospitals and critical infrastructure sites will face their own unique challenges.
To get a large amount of high-quality data for AI training, companies have a few options. They can gather data from their own activities and make their current data better with special techniques. They can work with others to buy data or use data that is freely available. They can ask people to share their data. Whatever alternative is selected, making sure the data is clean and well organized is critical. Also, using existing AI models that have already “learned” some things can mean that less data will be required.
AI can significantly improve efficiency, predictive analytics and response times. However, the quality and maintenance of existing systems play a crucial role in determining the technology’s effectiveness. The planning and deployment of AI systems requires multiple factors to be considered.
- Use of existing infrastructure: It is often feasible to use existing systems as a foundation for implementing AI solutions. Many AI tools and algorithms are designed to integrate with current infrastructure, processing and analyzing the collected data to provide insights, detect anomalies, or predict potential security breaches.
- Data quality and maintenance: The effectiveness of AI is heavily dependent on the quality of data. Systems with issues like frequent false alarms or poorly maintained sensors can generate noisy data, leading to inaccurate AI predictions and anomaly detection. If an organization has not sufficiently maintained its security system, this noise can significantly undermine the performance of AI applications.
- System compatibility: Older systems may not be fully compatible with modern AI technologies, limiting the potential benefits or requiring substantial middleware development to facilitate integration.
- Assessment and cleanup: Before integrating AI, it is critical to conduct a thorough assessment of the current security system. Identifying and rectifying issues like false alarms, sensor malfunctions, or outdated software can improve the quality of data fed into AI systems.
- Incremental modernization: Completely overhauling the infrastructure may not be feasible, especially for large systems. An incremental approach – prioritizing upgrades that significantly affect AI performance, such as improving sensor quality or updating to more compatible systems – can be a more manageable strategy.
- Continuous monitoring and maintenance: Ongoing maintenance of the physical security infrastructure and continuous monitoring of AI system performance are essential. This approach allows for timely adjustments and ensures that AI applications remain effective and reliable.
- AI training and calibration: Training AI models on the specific dataset of the organization, including data from faulty alarms, can help the system learn to identify and possibly ignore these anomalies over time. Continuous calibration based on real-world performance can further refine AI accuracy.
- IT infrastructure complexity: AI systems add layers of complexity to IT infrastructures, complicating data protection consistency across platforms.
- Regulatory compliance: The dynamic nature of AI challenges compliance with evolving regulations, necessitating constant vigilance.
- Cybersecurity threats: AI systems require the implementation of sophisticated, dynamic defenses to protect against increasingly smart cyber threats.
- Technical skill shortages: The specialized knowledge required for AI data protection and the shortage of skilled professionals can impede effective strategy execution.
- Budget constraints: The ability to implement cutting-edge AI security solutions is often limited by the availability of funds, affecting the quality of deployed measures.
- Data sprawl: The rapid growth and dispersion of data generated by AI systems challenge efforts to maintain control and visibility.
- Ensuring reliability: Updating AI systems with the latest security measures without compromising their reliability or performance poses a significant challenge.
While existing physical security systems can form the basis for AI integration, ensuring the quality and compatibility of these systems is crucial. Through careful assessment, incremental modernization and ongoing maintenance, AI can provide reliable, efficient enhancements to security operations.