Predictive Analytics: A Proactive Security Solution
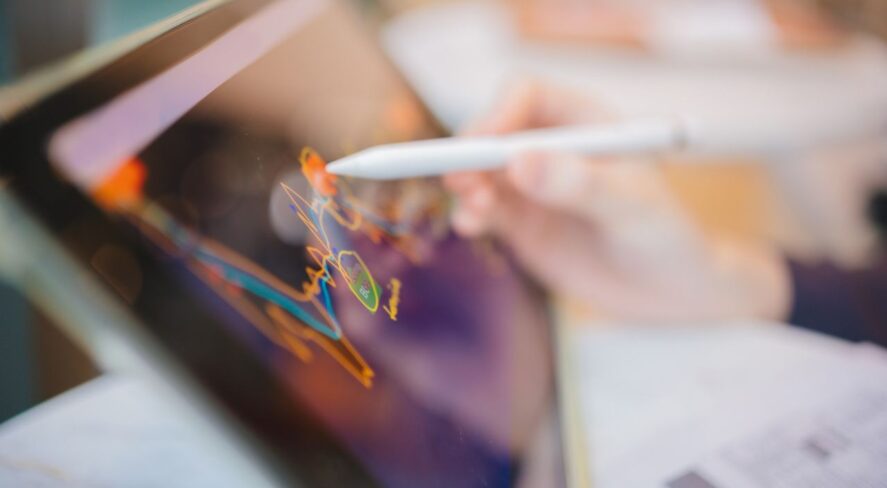
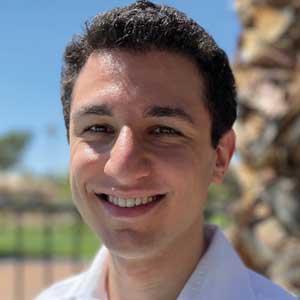
The physical security industry is being transformed by moving from being reactive to proactive, thanks to the integration of artificial intelligence (AI) and predictive analytics.
The introduction of AI is making surveillance systems smarter, more efficient and prognostic.
Threat + Anomaly Detection
AI works by using machine-learning algorithms to detect unusual behavior. Unlike human staff, AI can digest an enormous amount of data at once, including video feeds, flagged events, movements and patterns. After taking in this information, the algorithm “learns” how to interpret “normal” occurrences versus “abnormal” events.
For example, if a camera suddenly becomes blocked or blurry, AI would immediately flag this as an anomaly and raise an alert to the operator. Other security threats could be flagged as well, such as loitering, large crowds, fires or even unauthorized entry. Such a system can send alerts in real time to security staff, drastically increasing response time.
Prediction and Prevention
While AI algorithms can detect and flag unusual behavior in real time, predictive analytics take this technology one step further. Predictive models can identify high-risk areas and forecast where security incidents are most likely to occur. Business owners can then build their physical security strategy and take measures to eliminate threats. Examples would be repairing a damaged entry point or deploying a guard to augment security at specific times in a high-risk area.
Resource and Operational Efficiency
Finally, AI and predictive analytics converge by enhancing maintenance and uptime and improving the overall efficiency of security systems.
When a camera goes down, AI can immediately alert users to the issue. Predictive models can also analyze metrics like usage and environment to forecast which cameras are most likely to fail at what times. As a result, service calls for repairs can be carried out quickly.
Instead of employing staff to monitor cameras around the clock, predictive AI gets the job done more efficiently. AI can reduce false alarms since it is quite good at distinguishing between a security threat and benign movements (animals, weather changes, etc.).
Implementation Challenges
A common use of AI in video surveillance is identifying objects and events (i.e., targets). While a person may be able to identify targets from any vantage point or in any lighting conditions, AI is typically more limited and often requires the camera to be positioned at a specific height, distance and angle relative to the intended target – and with adequate lighting – for optimal accuracy.
Examples include detecting whether a person has crossed a line. If the camera is moved or repositioned, it may be unable to detect targets accurately since a previously configured “line” may now be somewhere entirely different in the view. Similarly, view degradation, such as occlusion, blurriness or lighting changes, can diminish effectiveness. Something as innocuous as a flashlight or spotlight can cause a glare and degrade AI’s accuracy.
The views and opinions expressed in guest posts and/or profiles are those of the authors or sources and do not necessarily reflect the official policy or position of the Security Industry Association.
This article originally appeared in All Things AI, a newsletter presented by the SIA AI Advisory Board.