What Artificial Intelligence Means for Physical Security
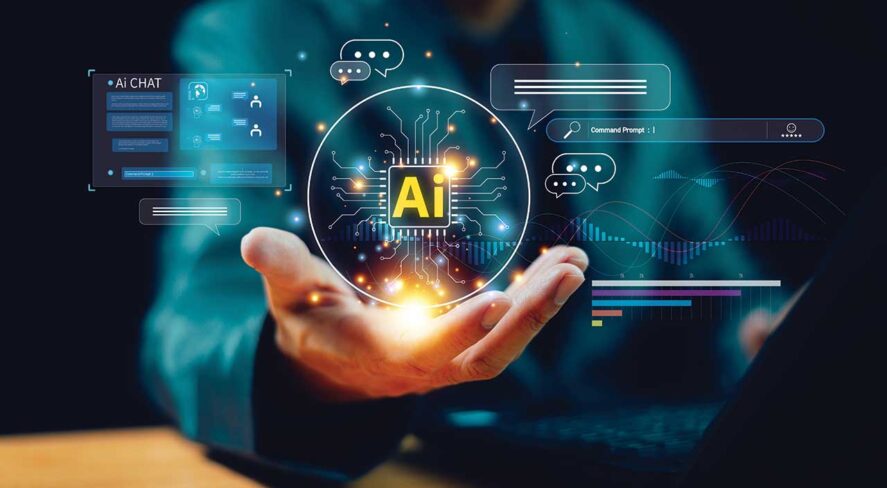
Managing risk is essential to leveraging this emerging technology
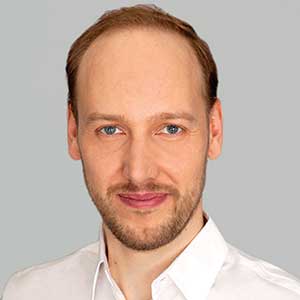
Large language models (LLMs) have recently taken the world by storm. Only months after OpenAI launched its artificial intelligence (AI) chatbot, ChatGPT, it amassed more than 100 million users. This makes it the fastest-growing consumer application in history.
And there is little wonder why. LLMs can do everything from answering questions and explaining complex topics to drafting full-length movie scripts and even writing code. Because of this, people everywhere are both excited and worried about the capabilities of this technology.
Although LLMs have recently become a hot topic, it is worth noting that the technology has been around for a long time. With advancements underway, however, LLMs and other AI tools are creating new opportunities to drive greater automation across various tasks. Having a grounded understanding of AI limitations and potential risks is essential.
Clarifying the Terminology
Artificial intelligence, machine learning, deep learning and other terms are often discussed, but what are the differences?
- Artificial intelligence: The concept of simulating human intelligence through machines. It refers to tools and processes that enable machines to learn from experience and adjust to new situations without explicit programming. In a nutshell, machine learning and deep learning both fall into the category of artificial intelligence.
- Machine learning: AI that can automatically learn with little human involvement.
- Deep learning: A subset of machine learning that uses artificial neural networks that learn based on large amounts of data.
- Natural language processing: The process of using AI and machine learning to understand human language and automatically perform repetitive tasks such as spellcheck, translation and summarization.
- Generative AI (gen-AI): Enables users to quickly generate content based on a variety of inputs, such as text and voice, resulting in outputs in the form of images, video and other types of data.
- LLM: A type of gen-AI that can perform natural language processing and is trained on vast amounts of data.
Artificial Intelligence vs. Intelligent Automation
Automation is when tasks, whether easy or hard, are done without the involvement of a person. Once a process is set up in a program, it can repeat itself whenever needed, always producing the same result.
Traditional automation requires a clear definition from the start. Every aspect, from input to output, must be carefully planned and outlined by a person. Once defined, the automated process can be triggered to operate as intended.
Intelligent automation (IA) allows machines to tackle simple or complex processes, without these processes needing to be explicitly defined. IA typically uses gen-AI and natural language processing to suggest ways to analyze data or take actions based on existing data and usage patterns.
Risks of Large Language Models
When weighing the risks of LLMs, it is important to consider that LLMs are trained to satisfy the user as their first priority. LLMs also use an unsupervised AI training method to feed off a large pool of random data from the Internet. This means the answers they give are not always accurate, truthful or bias-free. All of this can become dangerous in a security context.
This unsupervised AI method has opened the door to what are now called “hallucinations,” which occur when an AI model generates answers that seem plausible but are not factual or based on real-world data.
Using LLMs can also create serious privacy and confidentiality risks. The model can learn from data that contain confidential information about people and companies. And since every text prompt is used to train the next version, someone prompting the LLM about similar content might become privy to sensitive information through AI chatbot responses.
Then there are the malicious abuses of this AI technology. Consider how bad actors with little or no programming knowledge could ask an AI chatbot to write a script that exploits a known vulnerability or provide a list of ways to hack specific applications or protocols. One cannot help but wonder how these technologies could be exploited in ways that have not yet been anticipated.
Leveraging AI in Physical Security
AI-enabled applications are advancing in new and exciting ways. They show great promise in helping organizations achieve specific outcomes that increase productivity, security and safety.
One of the best ways to capitalize on AI advances in physical security is by implementing an open security platform. Open architecture gives security professionals the freedom to explore AI applications that drive greater value across their operations. As AI solutions come to market, leaders can try out these applications, often for free, and select the ones that best fit their objectives and environment.
As new opportunities emerge, so do new risks. That is why it is important to partner with organizations that prioritize data protection, privacy and the responsible use of AI. This will not only help enhance cyber resilience and foster greater trust in an organization, it is also part of being socially responsible.
Since AI algorithms can process large amounts of data quickly, AI is becoming an increasingly important tool for physical security solutions. But as AI evolves, it greatly expands the risk of using personal information in ways that can intrude on privacy. The three pillars below can provide guidance when developing or evaluating AI solutions.
Privacy and data governance
Only use datasets that respect relevant data protection regulations. Wherever possible, ethically source, anonymize and securely store data used for training machine learning models. Treat datasets with the utmost care and keep data protection and privacy top of mind. This includes sticking to strict authorization and authentication measures to ensure the wrong people do not get access to sensitive data and information across AI-driven applications.
Trustworthiness and safety
When developing and using AI models, always think about how to minimize bias. Ensure that AI models are rigorously tested and that accuracy is continuously improved. Finally, make sure AI models are easily explainable. When AI algorithms deliver an outcome, one should be able to see exactly how it reached that conclusion.
Humans in the loop
AI models cannot make critical decisions on their own. A human should always have the final say. In a physical security context, prioritizing human-centric decision-making is critical. Machines simply cannot grasp the intricacies of real-life events like a security operator, so relying solely on statistical models is not an option. Systems should always drive insights to enhance human capacity for judgment.
AI models can inadvertently produce skewed decisions or results based on various biases. This can affect decisions and ultimately lead to discrimination. While AI has the power to revolutionize how work in the security industry and beyond is done and how decisions are made, it needs to be deployed responsibly.