Making the Most of Edge AI in the Security Industry
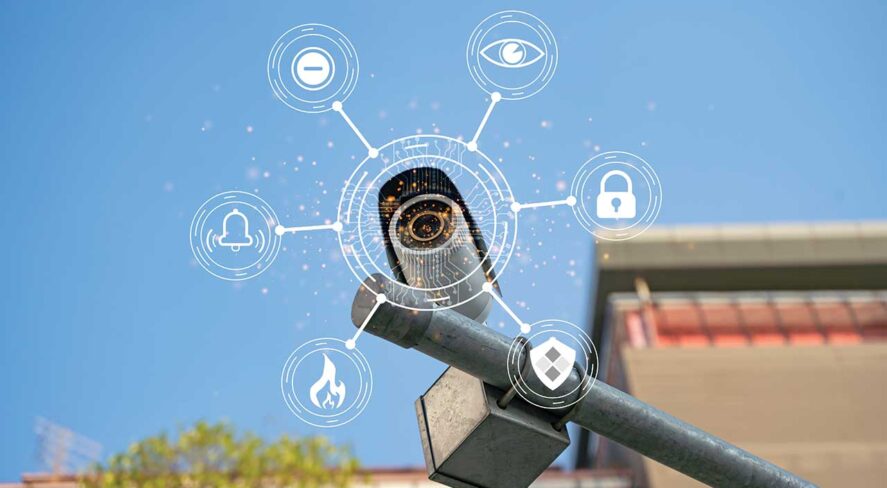
Edge monitoring is key to system reliability and data integrity
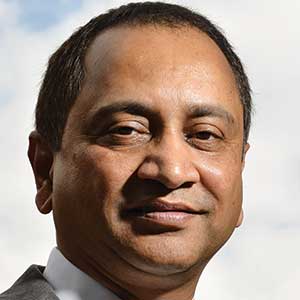
The rapid development of artificial intelligence (AI) and machine learning (ML) and the proliferation of connected devices have combined to fundamentally change how businesses and industries operate.
AI/ML is no longer confined to cloud data centers. It is now shifting toward the edge, enabling real-time intelligence from and on local devices and networks. This transformation is revolutionizing the security industry, enhancing everything from surveillance and access control to threat detection and emergency response.
As organizations look to harness edge AI, one crucial element that cannot be overlooked is edge monitoring – the ability to track, manage and optimize distributed compute efficiently. For security applications, edge monitoring ensures operational reliability, data integrity and real-time responsiveness to potential threats.
Understanding Edge AI and Why It Matters for Security
Edge AI refers to the deployment of ML models directly on edge devices or local networks, as opposed to relying on centralized cloud infrastructure. It also refers to using the edge data to drive a two-way, closed loop system: Read → Analyze → Automate (back).
This approach offers several key advantages for the security industry.
- Low Latency: Since data is processed at the edge itself or a nearby edge node, there is minimal delay in decision-making, which is critical for applications like real-time surveillance, intrusion detection and law enforcement.
- Privacy and Security: By keeping sensitive data localized, edge AI minimizes exposure to cybersecurity threats, reducing compliance risks and providing better control over confidential information. Of course, the distributed components need to be monitored, patched etc., for good cyber hygiene.
- Bandwidth Optimization: Transmitting large amounts of security footage and sensor data to the cloud for processing can be expensive and inefficient. Edge AI minimizes bandwidth costs by processing essential data locally and only sending relevant alerts to security teams.
- Resilience and Reliability: Edge AI enables continued operations even in environments with limited or no Internet connectivity, making it ideal for remote security monitoring, smart surveillance systems, and mission-critical applications.
The convergence of low-latency connectivity, next-generation networks (5G), and more efficient AI models is making it possible for businesses to push intelligence closer to where data is generated, unlocking unprecedented innovation in security and public safety.
Competitive Advantages at the Edge
As the security industry continues to evolve, edge (and ambient) intelligence will become the backbone of modern surveillance and protection systems. Organizations that embrace edge AI will gain competitive advantages by improving response times, reducing costs and enhancing security operations.
However, to unlock the full potential of edge AI, robust edge monitoring solutions are essential. By providing real-time visibility, automation and resilience to edge deployments, security teams can ensure that AI-powered surveillance and monitoring systems remain effective and reliable.
The coming years are likely to bring advances in AI-powered threat detection, federated learning for security, and enhanced cybersecurity frameworks to further accelerate the adoption of edge intelligence in security. Businesses that proactively invest in edge AI and edge monitoring today will be well-positioned to lead in the future of security technology.
The Need for Edge Monitoring in Security
While edge AI offers transformative benefits, it also introduces new challenges. Managing a distributed AI/ML infrastructure requires continuous edge monitoring to ensure performance, security and compliance. Without real-time visibility into the health of edge devices, security teams risk inefficiencies, security breaches and system failures.
Edge monitoring allows organizations to:
- Track the health, performance and uptime of security devices and associated infrastructure
- Detect and resolve potential system failures before they escalate
- Ensure compliance with security and data governance policies
- Optimize edge components’ performance and resource utilization
- Provide remote diagnostics and predictive maintenance for security systems
Effective edge monitoring allows businesses to move beyond reactive troubleshooting to proactive threat detection, reducing downtime and improving operational efficiency.
Real-World Applications
AI-powered edge surveillance for smart cities
Urban centers are increasingly deploying surveillance cameras with embedded edge AI to monitor streets, public spaces and transportation hubs. These cameras process video feeds locally, monitoring traffic and public services while also identifying suspicious activities such as unauthorized gatherings, unattended objects, unusual movement patterns and other potential threats. Edge monitoring ensures that these systems remain fully operational, identifying hardware failures, network disruptions and storage limitations before they affect security operations. This approach enhances real-time situational awareness for law enforcement and emergency response teams.
Predictive equipment maintenance for critical security infrastructure
Security infrastructure includes a vast range of critical equipment, from biometric scanners and security gates to alarm systems and access control panels. Edge AI enables real-time monitoring of these devices, collecting performance data and detecting anomalies that indicate potential failures. Predictive maintenance powered by edge AI helps security teams proactively service equipment before breakdowns occurs, ensuring operational continuity. Edge monitoring solutions track device health, alert technicians to irregularities, and automate diagnostics to maintain seamless security coverage.
Real-time perimeter security with smart sensors
Perimeter security is evolving with AI-powered smart sensors that detect motion, vibrations and unauthorized entry based on object recognition. These sensors leverage edge AI to differentiate between harmless disturbances (e.g., animals, weather conditions) and genuine threats. Edge monitoring plays a crucial role in maintaining sensor accuracy, ensuring seamless communication between devices and preventing security blind spots.
AI-driven access control systems
Facial recognition, biometric authentication, and RFID-based access control systems are widely used in high-security areas. Edge AI enables instant identity verification at entry points, eliminating the need for cloud-based authentication. Edge monitoring ensures that access control systems remain secure, continuously updated and free from operational disruptions that could compromise facility security.
Conclusion
The shift from cloud-centric AI to edge AI is not just a technological evolution, it is a paradigm shift that is redefining privacy, security and real-time intelligence. With the right edge monitoring strategies and solutions, organizations can fully capitalize on this transformation while mitigating risks associated with distributed AI deployments.
As AI scales from the cloud to the edge, the ability to manage, monitor and optimize distributed intelligence will determine the success of security innovations. Organizations that take a proactive approach to edge monitoring and intelligence orchestration will lead the next wave of smart security and AI-driven protection systems.